Abstract
Rabbit breed classification poses challenges due to the diverse physical characteristics and colour patterns, complicating differentiation, especially for untrained individuals. Misclassification can detrimentally affect mating, genetic selection, and rabbit health. Thus, developing an accurate classification system is crucial for the breeding and farming industry. This project aims to study the CNN algorithm's efficacy in rabbit breed classification, develop a CNN-based prototype, and evaluate its accuracy. Four widely raised rabbit breeds in Malaysia—Californian, Holland Lop, Lionhead, and New Zealand—are selected for classification due to their distinct characteristics. The research methodology encompasses preliminary study, design and implementation, and evaluation phases. Literature review, knowledge acquisition, and dataset collection inform algorithm selection and dataset validation. The design and implementation phase focus on prototype development, while the evaluation phase assesses classification performance. Results demonstrate the CNN algorithm's potential for achieving high accuracy, with an average accuracy of 95%. This study underscores the CNN algorithm's viability for accurate rabbit breed identification. It concludes by recommending further research into its application in other animal classification contexts, highlighting broader implications.
Metadata
Item Type: | Thesis (Degree) |
---|---|
Creators: | Creators Email / ID Num. Mohd Zaid, Aishah Nabila 2022900667 |
Contributors: | Contribution Name Email / ID Num. Thesis advisor Raju, Rajeswari UNSPECIFIED |
Subjects: | Q Science > QA Mathematics > Instruments and machines > Electronic Computers. Computer Science > Neural networks (Computer science) |
Divisions: | Universiti Teknologi MARA, Terengganu > Kuala Terengganu Campus |
Programme: | Bachelor of Computer Science (Hons) |
Keywords: | Rabbit breed, CNN Algorithm's |
Date: | 2024 |
URI: | https://ir.uitm.edu.my/id/eprint/95549 |
Download
![[thumbnail of 95549.pdf]](https://ir.uitm.edu.my/style/images/fileicons/text.png)
95549.pdf
Download (78kB)
Digital Copy
Physical Copy
ID Number
95549
Indexing
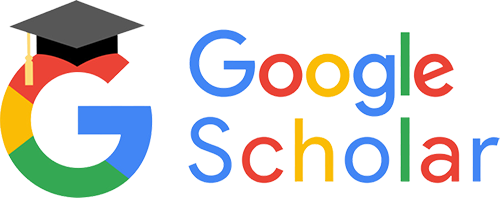
