Abstract
This study addresses the communication challenges faced by the deaf and hard of hearing community in Malaysia due to the scarcity of Malaysian Sign Language (MSL) interpreters. MSL, as the official sign language of the country, plays a crucial role in facilitating communication for individuals with hearing impairments. However, the limited availability of interpreters hinders effective communication. In response, this paper introduces an innovative approach utilizing Long Short-Term Memory (LSTM) neural networks for the recognition of Malaysian Sign Language. LSTM networks, recognized for their proficiency in sequence modeling tasks, are employed to discern the temporal correlations inherent in the hand movements constituting MSL signs. The proposed method is rigorously evaluated using a dataset comprised of MSL signs, incorporating metrics such as accuracy and confusion matrix analysis. The results demonstrate a notable precision in sign recognition, reinforcing the potential of the method to form the foundation for a real-time MSL recognition system. By leveraging the capabilities of LSTM networks, this research contributes to enhancing accessibility and communication for the deaf and hard of hearing community in Malaysia.
Metadata
Item Type: | Thesis (Degree) |
---|---|
Creators: | Creators Email / ID Num. Mohamad Nizam, Nurul Amila Ilyana 2022736255 |
Contributors: | Contribution Name Email / ID Num. Thesis advisor Othman, Ahmad Kamarulzaman UNSPECIFIED |
Subjects: | Q Science > QA Mathematics > Instruments and machines > Electronic Computers. Computer Science > Neural networks (Computer science) |
Divisions: | Universiti Teknologi MARA, Terengganu > Kuala Terengganu Campus |
Programme: | Bachelor of Computer Science (Hons) |
Keywords: | Long Short-Term Memory (LSTM), Malaysian Sign Language (MSL) |
Date: | 2024 |
URI: | https://ir.uitm.edu.my/id/eprint/96519 |
Download
![[thumbnail of 96519.pdf]](https://ir.uitm.edu.my/style/images/fileicons/text.png)
96519.pdf
Download (93kB)
Digital Copy
Physical Copy
ID Number
96519
Indexing
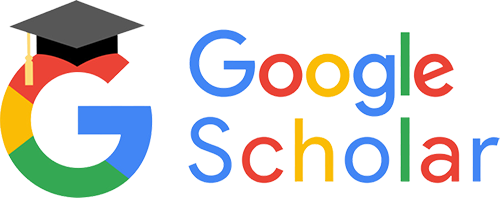
