Abstract
This project delves into the design and implementation of a predictive model aimed at forecasting bank customer churn, employing the Random Forest algorithm. In the dynamic landscape of the banking industry, the phenomenon of customer churn presents a formidable challenge. This study seeks to confront and mitigate this challenge by harnessing the efficacy of Random Forest, a machine learning ensemble technique renowned for its resilience and precision. To achieve this, the research involves the meticulous collection of diverse customer data, encompassing transaction histories, demographic details, and various customer interactions. The data is utilized to train and validate the Random Forest model, with a comprehensive evaluation of its predictive performance metrics such as accuracy, precision, recall, and F1-score. Notably, the Random Forest model achieved an accuracy score of 79.97%. The anticipated outcomes of this research not only contribute to the progression of predictive modeling within the banking sector but also furnish financial institutions with actionable insights to proactively discern and address customer churn.
Metadata
Item Type: | Thesis (Degree) |
---|---|
Creators: | Creators Email / ID Num. Rozi, Nur Iffah Malihah 2022736391 |
Contributors: | Contribution Name Email / ID Num. Thesis advisor Syed Yasin, Sharifah Nurulhikmah UNSPECIFIED |
Subjects: | Q Science > QA Mathematics > Instruments and machines > Electronic Computers. Computer Science > Algorithms |
Divisions: | Universiti Teknologi MARA, Terengganu > Kuala Terengganu Campus |
Programme: | Bachelor of Computer Science (Hons) |
Keywords: | Predictive Model, Bank Customer Churn, Random Forest Algorithm |
Date: | 2024 |
URI: | https://ir.uitm.edu.my/id/eprint/96045 |
Download
![[thumbnail of 96045.pdf]](https://ir.uitm.edu.my/style/images/fileicons/text.png)
96045.pdf
Download (88kB)
Digital Copy
Physical Copy
ID Number
96045
Indexing
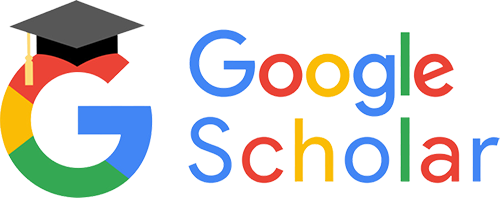
