Abstract
Effective detection of diseases in aquaculture is important for maintaining fish populations and encouraging appropriate practices. Traditional approaches frequently depend on visual inspection without any tools which can be difficult in terms of precision and productivity. This study presents a fish detection system that uses Convolutional Neural Networks (CNNs) and advanced image processing techniques, with a flexible research approach directing the iterative development process. The CNN model, chosen by algorithmic analysis, shows an impressive accuracy of 90% in automatically recognizing and diagnosing different fish diseases. By being trained on several datasets, the model can identify important features from images of fish. A program that is easy to use is then created for aquaculture professionals, allowing for quick and accurate disease diagnosis. This method represents a notable advancement in utilizing machine learning for disease control in aquaculture, surpassing the limitations of manual observation and contributing to the sustainable future of fish farming industries.
Metadata
Item Type: | Thesis (Degree) |
---|---|
Creators: | Creators Email / ID Num. Azahar, Nur Adriana Qaisara 2022901011 |
Contributors: | Contribution Name Email / ID Num. Thesis advisor Abdul Hamid, Nor Hasnul Azirah UNSPECIFIED |
Subjects: | Q Science > QA Mathematics > Instruments and machines > Electronic Computers. Computer Science > Neural networks (Computer science) |
Divisions: | Universiti Teknologi MARA, Terengganu > Kuala Terengganu Campus |
Programme: | Bachelor of Computer Science (Hons) |
Keywords: | Fish Population, Fish Detection System, Convolutional Neural Networks (CNNs) |
Date: | 2024 |
URI: | https://ir.uitm.edu.my/id/eprint/96037 |
Download
![[thumbnail of 96037.pdf]](https://ir.uitm.edu.my/style/images/fileicons/text.png)
96037.pdf
Download (90kB)
Digital Copy
Physical Copy
ID Number
96037
Indexing
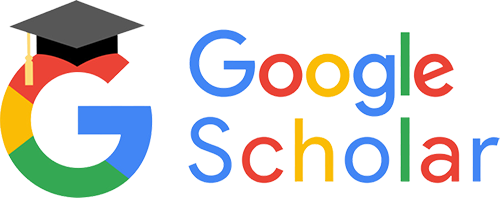
