Abstract
Predicting a corporate default is essential to avoid failure in business operations. Default is said as an unpredicted event that may cause a failure in paying debts on time. This study aims to predict the default and non-default of corporations using Decision Tree (DT), Random Forest (RF) and Gradient Boosted (GB) models. Not only that, the best models used in predicting the corporate default are determined by using cross validation on the models performance evaluation (classification). A sample of financial data of 24 Malaysian companies consisting of companies listed as Practice Note 17 (PN17) and main market companies in the Bursa Malaysia board is used. The data is utilized to estimate the prediction of the companies by using the KMV-Merton model. It is also used to calculate variables such as Net Profit Margin, Cash Flows, Cash Coverage Ratio, Current Ratio, GDP, Currency Rates and Volatility. Then, analysis is done on the dependent and independent variable by using RapidMiner Studio Educational software. As a result, the accuracy of RF is 84.17% which is the highest followed by the accuracy of GB and DT is 79.17% and 75.83% respectively. Therefore, RF is the best model to use for predicting default and non-default companies. This study encourages increasing the use of machine learning models in default forecasting. Thus, it is recommended to increase more data based on the most relevant independent variables that are used in the study. Then, by using a combination of different machine learning models and software can yield a better performance and improve the overall effectiveness of the models.
Metadata
Item Type: | Student Project |
---|---|
Creators: | Creators Email / ID Num. Shamsul, Nur Sabrina UNSPECIFIED Ahmad Shah, Fayyadh Izzuddin UNSPECIFIED Ridzuan, Nurul Zahidah UNSPECIFIED |
Subjects: | L Education > LB Theory and practice of education > Higher Education > Dissertations, Academic. Preparation of theses |
Divisions: | Universiti Teknologi MARA, Negeri Sembilan > Seremban Campus |
Programme: | Bachelor of Science (Hons.) (Mathematics) |
Keywords: | Decision tree, random forest, Cash Flows, Cash Coverage Ratio, Current Ratio, GDP, Currency Rates |
Date: | 2023 |
URI: | https://ir.uitm.edu.my/id/eprint/93504 |
Download
![[thumbnail of 93504.pdf]](https://ir.uitm.edu.my/style/images/fileicons/text.png)
93504.pdf
Download (94kB)
Digital Copy
Physical Copy
ID Number
93504
Indexing
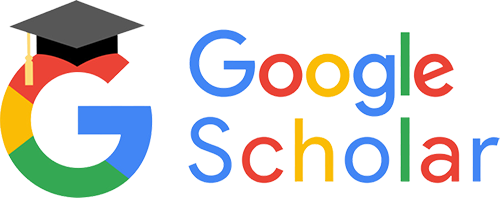
