Abstract
Autism spectrum disorder is one of those diseases that is not unfamiliar to our lives today. In contrast to healthier individuals, the disorder has some problems, such as being slow to talk. The development of Autism Spectrum Disorder in children is a key objective of this research. The Ant-Miner algorithm was then used in this analysis to establish classification rules for the prediction of autistic spectrum disorder. The Ant-Miner algorithm was used to predict the ASD and was compared with the J48 algorithm. The accuracy of Ant-Miner is 87.97%, while the accuracy of J48 is 72.68%. The result shows that Ant-Miner is better predicting accuracy than J48. This research also shows that ACO is an appropriate technique in the design of the classification model. In addition, the Ant-Miner algorithm is acceptable in this analysis because it can train data several times in order to achieve the maximum percentage of accuracy to predict the ASD for the development of the classification model. This research recommends that children continue to predict future autism spectrum disorders, which will increase the consistency of the model.
Metadata
Item Type: | Student Project |
---|---|
Creators: | Creators Email / ID Num. Zafri, Nur Ibtisyam 2019705213 |
Subjects: | Q Science > QA Mathematics > Mathematical statistics. Probabilities > Prediction analysis R Medicine > RJ Pediatrics > Diseases of children and adolescents |
Divisions: | Universiti Teknologi MARA, Perlis > Arau Campus > Faculty of Computer and Mathematical Sciences |
Programme: | Management Mathematics |
Keywords: | Predicting Autistic Spectrum Disorder ; Toddler ; Ant Colony Optimization ; ACO |
Date: | 5 August 2021 |
URI: | https://ir.uitm.edu.my/id/eprint/49378 |
Download
![[thumbnail of 49378.pdf]](https://ir.uitm.edu.my/style/images/fileicons/text.png)
49378.pdf
Download (456kB)
Digital Copy
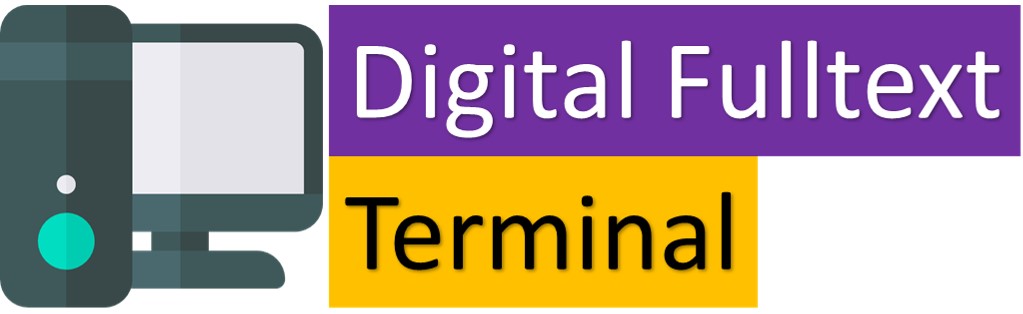
Physical Copy
ID Number
49378
Indexing
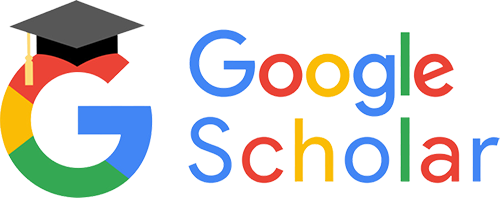
