Abstract
A predictive model was developed to provide a mechanism that can be regionally simulated, and predict low or high risk windstorm, its possible location, time, duration of the risk, intensity as well as potential damages. This development corresponds to the windstorm hazard monitoring mechanism which is not available in the country. The predictive model includes 16 prediction processes with 20 back-propagation algorithms whereby radar imageries and meteorological station data were used as a raw data input. Development of the predictive model is according to the most frequent cause of windstorm in the country, applicable observational data, existing severe weather monitoring system and a current widely used machinelearning model in forecasting. For the establishment of back-propagation algorithms, it does not only use Artificial Neural Network (ANN) model but also other techniques such as multiple regression analysis, dichotomous forecast method and error difference method. Besides, multiple correlation coefficient (R) between input units and output unit is set to be higher than 0.7, taking into consideration of atmospheric complexity and to avoid back-propagation algorithm from the poorly generated output that could be beyond the acceptable range since each prediction process relies on each other. Local conditions wind multiplier map, hazard threshold, and damage scales are three supporting tools that were developed to compliment the predictive model. The predictive model is highly accurate because the R-values for 14 prediction processes are higher than 0.7 ranging from 0.704 to 1.00. In addition, the mean square error (MSE) values for ANN model algorithms (pattern recognition tool) for 5 prediction processes are low from 0.00 to 0.0286 and errors from 0.00 to 0.0309. The other 11 prediction processes which utilised ANN model algorithms (fitting tool), gave R-values for all the algorithms higher than 0.900 except one algorithm equal to 0.8661, meanwhile MSE between 0.22 to 116.41. For verification, high Critical Success Index (CSI) and error difference whether in unit (minutes, km and m/s) or percentage were in acceptable range reinforced that the predictive model is able to simulate output at high precision. The CSI for 5 prediction processes are equal to 1.00 and error difference for 9 prediction processes are within ± 0.0 to ± 24.0 %. According to the error difference in unit, in terms of time for 6 prediction processes, the difference is between ± 0 to ± 5 minutes, distance for 2 prediction processes, the difference is between ± 1 to ± 4 km and the intensity for 1 prediction process is ± 1 m/s. Lead time is between 10 to 60 minutes. However, prediction process 5 and 16 are the only prediction processes need an attention for enhancement since the CSI average is low, 0.45 to 0.65 and 0.36 to 0.51 respectively. This is due to the missing and false number of grid area resulted from prediction, even though high hit number of grid area and the centroid distances between predicted convective cell area and actual area is short in range from 2.1 to 4.0 km.
Metadata
Item Type: | Thesis (PhD) |
---|---|
Creators: | Creators Email / ID Num. Bachok, Mohd Fatruz UNSPECIFIED |
Contributors: | Contribution Name Email / ID Num. Advisor Harun, Sobri (Prof. Dr.) UNSPECIFIED |
Subjects: | T Technology > TA Engineering. Civil engineering > Systems engineering T Technology > TA Engineering. Civil engineering > Mechanics of engineering. Applied mechanics |
Divisions: | Universiti Teknologi MARA, Shah Alam > Faculty of Civil Engineering |
Programme: | Doctor of Philosophy (Civil Engineering) |
Keywords: | Mechanism, Algorithms, Critical Success Index (CSI) |
Date: | 2019 |
URI: | https://ir.uitm.edu.my/id/eprint/45330 |
Download
![[thumbnail of 45330.pdf]](https://ir.uitm.edu.my/style/images/fileicons/text.png)
45330.pdf
Download (146kB)
Digital Copy
Physical Copy
ID Number
45330
Indexing
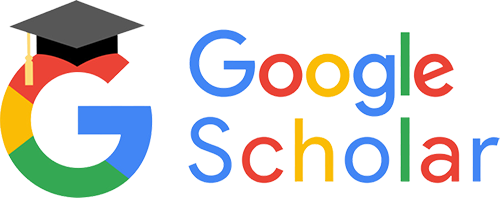
