Abstract
Deep learning methods that are the Convolution Neural Network can be utilized to classify the plant disease. In addition, Sliding Windows methods also help to create dataset in ease. This research will lead as one of future references in the modern agricultural sector. Plant disease has been identified as a significant threat to food security, as it significantly decreases crop yield and compromises its consistency classification as human in the existence of plant disease is. Manual detection is limited only to small-scale agriculture. Therefore, the automatic detection of crop diseases in the agricultural sector is very important as it will enable farmers to keep track of the underlying diseases from time to time. Therefore, the purpose of this project is sliding window is used to produce a dataset. The sliding window will help the image shifter to generate faster and larger datasets. Deep convolutional neural network is implemented in order to classify diseases through the use of a dataset of images of healthy plant leaves collected under controlled conditions using Matlab platform. In this research, a number of 8554 data of each leaf set with different angles and scales are used to perform the pre-train the dataset by using Convolutional Neural Network platform. The experimental results show a good precision of 94.81 percent of testing average result, suggesting as successful classification rate. This project also can make a farmers easily to classify the disease that affected their plants.
Metadata
Item Type: | Student Project |
---|---|
Creators: | Creators Email / ID Num. Zahari, Mohamad Lokman 2017668636 |
Contributors: | Contribution Name Email / ID Num. Thesis advisor Ismail, Ahmad Puad UNSPECIFIED |
Subjects: | T Technology > TK Electrical engineering. Electronics. Nuclear engineering > Electronics T Technology > TK Electrical engineering. Electronics. Nuclear engineering > Electronics > Detectors. Sensors. Sensor networks T Technology > TK Electrical engineering. Electronics. Nuclear engineering > Electronics > Computer engineering. Computer hardware T Technology > TK Electrical engineering. Electronics. Nuclear engineering > Electronics > Computer engineering. Computer hardware > Malaysia |
Divisions: | Universiti Teknologi MARA, Pulau Pinang > Permatang Pauh Campus > Faculty of Electrical Engineering |
Programme: | Bachelor of Engineering (Hons) Civil (Infrastructure) |
Keywords: | Convolution Neural Network, Sliding Window, Agriculture |
Date: | July 2020 |
URI: | https://ir.uitm.edu.my/id/eprint/44324 |
Download
![[thumbnail of 44324.pdf]](https://ir.uitm.edu.my/style/images/fileicons/text.png)
44324.pdf
Download (228kB)
Digital Copy
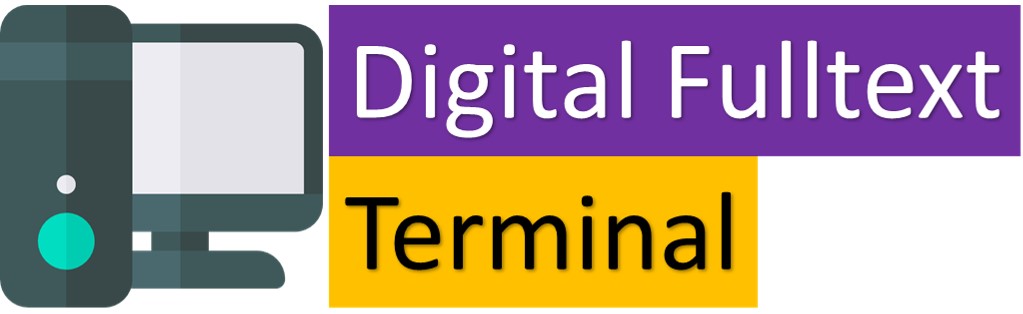

Physical Copy
ID Number
44324
Indexing
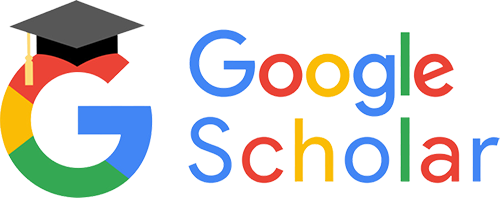
