Abstract
Diagnosis of power quality disturbances which include detection and classification of the disturbance is important before any further action can be carried out by both utility and customer. Fast and accurate techniques are required for detecting the disturbances so that proper mitigation of power quality problems can be implemented. The objective of this research is to classify two types of transient based on their unique characteristics in power system. Transient is the common type of power quality disturbance in the distribution system. Normally it can be caused by capacitor switching or lightning. In this research, two test systems have been used which is IEEE 13 bus system and IEEE 30 bus system for data generations. The simulations will be done using the PSCAD/EMTDC software. Switching of capacitors was simulated to generate oscillatory transients in a power distribution system. Lightning strikes model as in PSCAD library have been adapted to generate impulsive transient in this research. The disturbances waveforms obtained from the simulation axe decomposed by wavelet packet transform and used to extract the distinctive features of the voltage signals. Combination of Discrete Wavelet Transform (DWT) and Multiresolution Analysis (MRA) are used to decompose the signal of transient and to extract it useful information. Deubechies 4 (db4) was selected as a mother wavelet for the entire process in order to extract the features for the input of classifier. There are three different features or input to classifier in this research which are the maximum and minimum voltage, energy of the decomposed waveform and standard deviation combined with maximum voltages. After the feature extraction stage, Support Vector Machine (SVM) is used to classify the transient disturbance waveforms. In order to find the best analysis, two different kernels are used which are Gaussian RBF and Linear kernel. During classification, all the features are normalized to ensure it is properly scaled. Cross validation is used to find the best parameters related to kernels used followed by training and testing of the data sets. In this research, Multilayer perception (MLP) of feedforward Artificial Neural Network (ANN) has also been used as the classifier and comparison are made between the SVM and ANN method. The SVM results show that RBF kernel is superior to linear kernel in classifying the transient with average percentage of 83% This research has also proved that SVM has higher performance than the backpropagation algorithm of the ANN.
Metadata
Item Type: | Thesis (Masters) |
---|---|
Creators: | Creators Email / ID Num. Anuwar, Fahteem Hamamy 2008264862 |
Contributors: | Contribution Name Email / ID Num. UNSPECIFIED Hamzah, Noraliza (Assoc. Prof. Dr.) UNSPECIFIED |
Subjects: | T Technology > TJ Mechanical engineering and machinery > Mechanics applied to machinery. Dynamics T Technology > TJ Mechanical engineering and machinery > Control engineering systems. Automatic machinery (General) |
Divisions: | Universiti Teknologi MARA, Shah Alam > Faculty of Electrical Engineering |
Programme: | Master of Science |
Keywords: | Transient disturbance, Wavelet, Vector machine |
Date: | 2012 |
URI: | https://ir.uitm.edu.my/id/eprint/39595 |
Download
![[thumbnail of 39595.pdf]](https://ir.uitm.edu.my/style/images/fileicons/text.png)
39595.pdf
Download (2MB)
Digital Copy
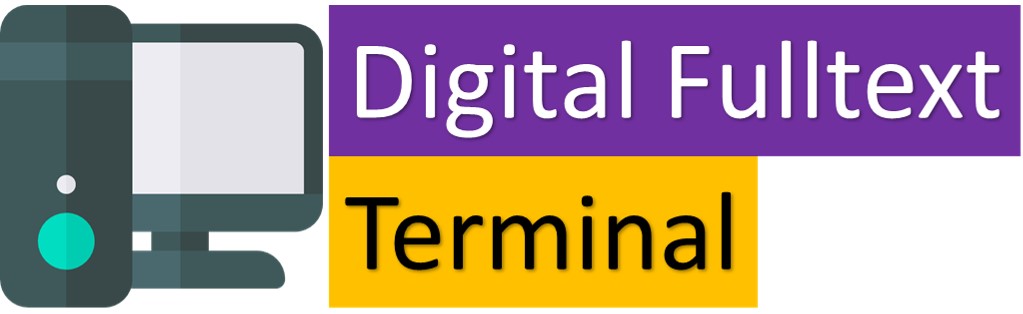
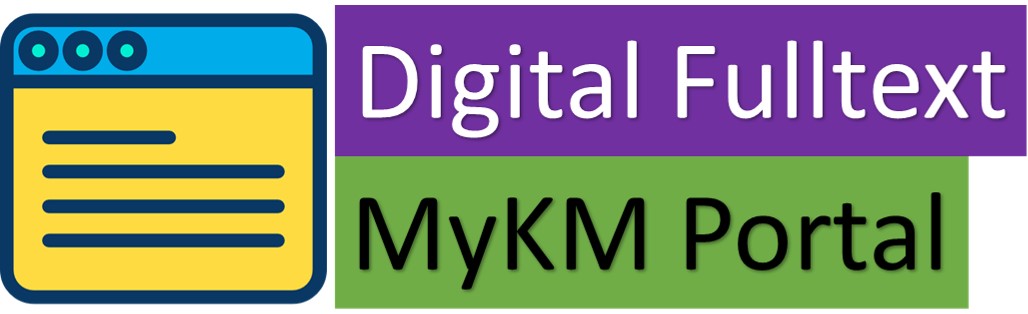
Physical Copy

ID Number
39595
Indexing
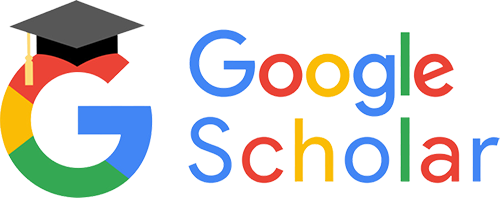
