Abstract
The coronavirus disease 2019 (COVID-19) outbreak urges authorities to manage pandemic outbreaks effectively. One of the most efficient ways to stop the COVID-19 virus from spreading is by requiring citizens to wear face masks properly. The fact that a portion of the face has been obscured makes the two-dimensional (2D) face recognition algorithm more challenging during the pandemic. Researchers proposed approaches to tackle these difficulties, such as a face-eye-based multi-granularity model, three-dimensional (3D) face reconstruction, synthesising a masked face and heterogeneous face recognition for deep neural networks. Nonetheless, 2D face recognition using deep neural networks to utilise 3D data requires converting data format, which results in the loss of geometric properties. The increasingly popular research of Deep Learning 3D face recognition using point clouds directly as input has produced promising results over the past few years. However, it required a lot of 3D point cloud data, which current 3D databases lacked, especially masked face databases. To address these problems, this study proposed a pipeline of works such as collected 3D masked face dataset and combined with the existing 3D dataset for train and evaluating networks, designed a triplet 3D face masked point clouds-aware deep network using triplet loss function, and evaluated the performance of 3D face recognition using Deep Learning model for identification of a person with a face mask. Experimentation has been carried out on the D415 3D face dataset (in-house dataset) and the Bosphorus 3D face dataset. A novel data augmentation using Poisson disk subsampling was implemented to enlarge the dataset. A triplet framework was proposed using the triplet loss function.
Metadata
Item Type: | Thesis (Masters) |
---|---|
Creators: | Creators Email / ID Num. Zuraimi, Muhammad Azhad 2021383913 |
Contributors: | Contribution Name Email / ID Num. Thesis advisor Mazlan, Lucyantie UNSPECIFIED |
Subjects: | T Technology > TA Engineering. Civil engineering > Applied optics. Photonics > Optical pattern recognition > Human face recognition (Computer science) |
Divisions: | Universiti Teknologi MARA, Shah Alam > College of Engineering |
Programme: | Master of Science (Electrical Engineering) |
Keywords: | 3D face recognition, dataset opens, triplet point ploud network |
Date: | 2023 |
URI: | https://ir.uitm.edu.my/id/eprint/106805 |
Download
![[thumbnail of 106805.pdf]](https://ir.uitm.edu.my/style/images/fileicons/text.png)
106805.pdf
Download (190kB)
Digital Copy
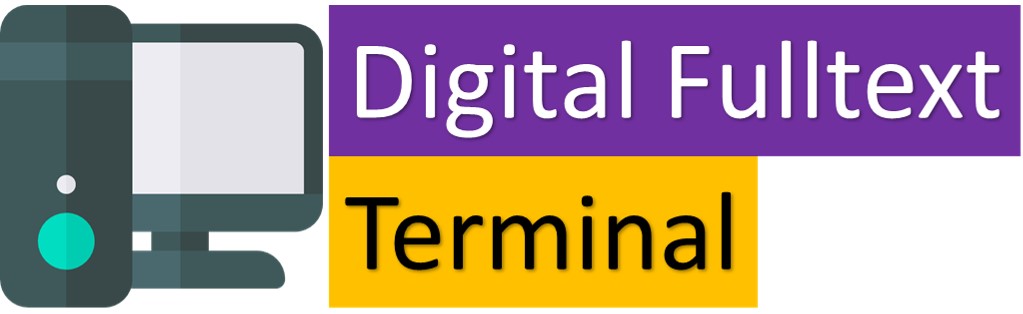
Physical Copy
ID Number
106805
Indexing
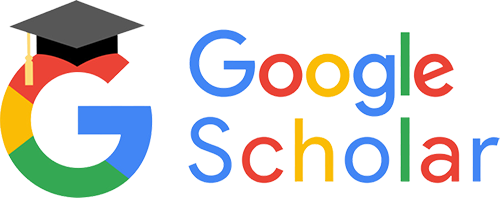
