Abstract
Skin cancer poses a significant health concern worldwide, emphasizing the need for effective early detection methods to enhance treatment outcomes and patient prognosis. Early detection of skin lesions is crucial as it increases the chances of identifying potentially cancerous growths, enabling timely intervention and improving overall treatment outcomes. Delayed detection may lead to advanced stages of disease, making it more challenging to treat successfully. Skin lesions can be classified as benign or malignant. Individuals with suspected skin lesions are strongly encouraged to consult healthcare professionals for a comprehensive evaluation. This study introduces the skin lesions detection system using convolutional neural network. The system was developed to detect the human skin whether it is normal or lesions skin. The system incorporates image preprocessing, including resizing and normalization, to enhance feature extraction. Utilizing the powerful CNN model known for its proficiency in learning hierarchical representations from image data, the system achieves an impressive accuracy of 98%.
Metadata
Item Type: | Thesis (Degree) |
---|---|
Creators: | Creators Email / ID Num. Mohd Arman, Anis Nadirah 2022758585 |
Contributors: | Contribution Name Email / ID Num. Thesis advisor Ismail, Habibah UNSPECIFIED |
Subjects: | Q Science > QA Mathematics > Instruments and machines > Electronic Computers. Computer Science > Neural networks (Computer science) |
Divisions: | Universiti Teknologi MARA, Terengganu > Kuala Terengganu Campus |
Programme: | Bachelor of Computer Science (Hons) |
Keywords: | Skin Lesions Detection System, Convolutional Neural Network |
Date: | 2024 |
URI: | https://ir.uitm.edu.my/id/eprint/96524 |
Download
![[thumbnail of 96524.pdf]](https://ir.uitm.edu.my/style/images/fileicons/text.png)
96524.pdf
Download (1MB)
Digital Copy
Physical Copy
ID Number
96524
Indexing
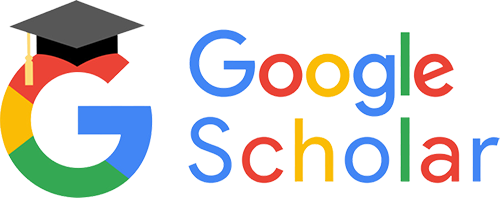
