Abstract
This project presents a study titled "Classification of Nutrient Deficiency in Lettuce using CNN." The research addresses challenges in diagnosing and categorizing nutrient deficiencies in lettuce, proposing a CNN-based solution to distinguish between nitrogen deficiency, phosphorus deficiency, potassium deficiency, and fully nutritional. The objectives involve investigating the requirements of CNN, developing a prototype system, and evaluating its accuracy. The system achieved a 92.68% accuracy in distinguishing between nitrogen deficiency, phosphorus deficiency, potassium deficiency, and fully nutritional. Chapter Two's literature review covers plant detection techniques and the advantages of CNN. Chapter Three outlines the methodology for CNN implementation, and Chapter Four presents the system's results and findings. Limitations include the absence of real-time detection and the inability to identify unknown images. Future recommendations aim to improve real-time detection, expand the range of nutrient deficient detection, and enhance accuracy through advanced algorithms.
Metadata
Item Type: | Thesis (Degree) |
---|---|
Creators: | Creators Email / ID Num. Mazlan, Mahirah 2022905871 |
Contributors: | Contribution Name Email / ID Num. Thesis advisor Tan, Gloria Jennis UNSPECIFIED |
Subjects: | Q Science > QA Mathematics > Instruments and machines > Electronic Computers. Computer Science > Neural networks (Computer science) |
Divisions: | Universiti Teknologi MARA, Terengganu > Kuala Terengganu Campus |
Programme: | Bachelor of Computer Science (Hons) |
Keywords: | Nutrient Deficiency, Convolutional Neural Network (CNN) |
Date: | 2024 |
URI: | https://ir.uitm.edu.my/id/eprint/95672 |
Download
![[thumbnail of 95672.pdf]](https://ir.uitm.edu.my/style/images/fileicons/text.png)
95672.pdf
Download (84kB)
Digital Copy
Physical Copy
ID Number
95672
Indexing
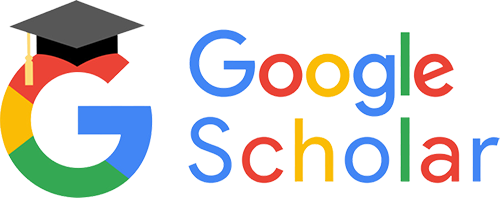
