Abstract
Weed detection and control are a focal point of agricultural research due to their harmful impact on crop productivity, competing for essential resources like sunlight, water, and nutrients. Modern agriculture recognizes weed detection systems as crucial tools to reduce the obstacles caused by weeds, enhancing crop growth and yield. This project aims to develop a weed detection prototype specifically for agricultural settings by utilizing Convolutional Neural Networks (CNN) algorithm. The project makes a thorough analysis and optimization of CNN hyperparameters in order to improve accuracy and efficiency. As a result, this study has achieved 89.82% from the 80-20 split using CNN algorithm with an F1 score of 88.08%. The research then goes on to assess how well the CNN model generalizes to various agricultural environments that support multiple crop situations. In addition to the technological innovations in agricultural technology, this CNN-based weed detection system proves to be a reliable resource for agriculturalists. It provides accurate and timely insights, empowering efficient weed control practices and contributing to the overall enhancement of agricultural processes.
Metadata
Item Type: | Thesis (Degree) |
---|---|
Creators: | Creators Email / ID Num. Mohammad Nasir, Khairun Nisa 2022930669 |
Contributors: | Contribution Name Email / ID Num. Thesis advisor Mohamed@Omar, Hasiah UNSPECIFIED |
Subjects: | Q Science > QA Mathematics > Instruments and machines > Electronic Computers. Computer Science > Neural networks (Computer science) |
Divisions: | Universiti Teknologi MARA, Terengganu > Kuala Terengganu Campus |
Programme: | Bachelor of Computer Science (Hons) |
Keywords: | Weed Detection, Convolutional Neural Network (CNN) Algorithm |
Date: | 2024 |
URI: | https://ir.uitm.edu.my/id/eprint/95565 |
Download
![[thumbnail of 95565.pdf]](https://ir.uitm.edu.my/style/images/fileicons/text.png)
95565.pdf
Download (79kB)
Digital Copy
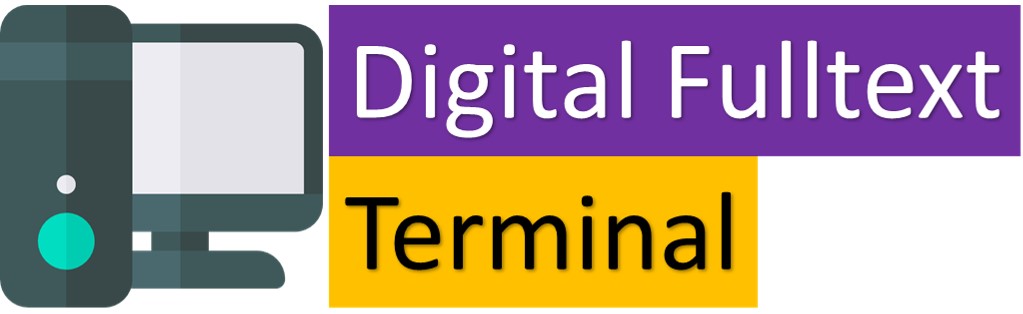

Physical Copy
ID Number
95565
Indexing
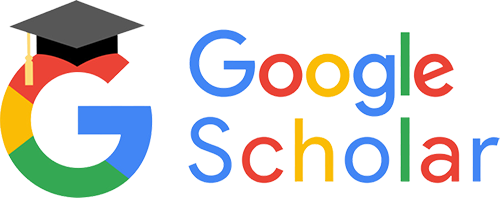
