Abstract
Exercise and repetitive movement fatigue are crucial to healthcare and rehabilitation. Muscle fatigue is a frustrating problem that happens after exercise. To improve the quality of rehabilitation and healthcare, precise evaluations and focused interventions are needed. Potential sites for force production can now be assessed using non-invasive stimulation approaches which is surface Electromyography (sEMG). Electromyography (EMG) is an essential technique used to measure muscle activity during various tasks. Despite its popularity, previous studies have yet to determine which EMG features are critical in classifying muscle contraction patterns across different physical activities and obtaining optimal performance. Therefore, this study aims to classify muscle contraction patterns during stepping exercises from sEMG and investigate the performance of Artificial Neural Networks as a classifier of this contraction pattern. 60 participants voluntarily participated in this exercise and their EMG signal was recorded. This study has been approved by the University of Birmingham Research Ethics Committee (ERN_14-0493). The raw EMG signal undergoes filtering, rectification, and linear enveloping. Then, each signal from each participant was segmented into a relax signal and a contract signal. Time domain and frequency domain features were then retrieved from every relax and contract signal and fed into the ANN model. For validation purposes, ANN model performance has been compared with other machine learning models which are support vector machines and Decision Tree. The results indicate that ANN outperformed the other machine learning models with 96.5% accuracy performance in classifying the muscle contraction patterns from the sEMG signal. The findings from this research could contribute valuable insights to inform more effective and personalized rehabilitation services in identifying muscle contractions autonomously, thereby improving the overall mobility and quality of life for individuals facing muscle fatigue and financial problems. This study could help in strengthening the healthcare services mentioned in Ekonomi Madani’s 17-Big Bolds.
Metadata
Item Type: | Student Project |
---|---|
Creators: | Creators Email / ID Num. Mazlan, Aiman Syabil UNSPECIFIED Suhaizan, Sofea Nur Batrisyia UNSPECIFIED Hishamudin, Zariq UNSPECIFIED |
Subjects: | L Education > LB Theory and practice of education > Higher Education > Dissertations, Academic. Preparation of theses |
Divisions: | Universiti Teknologi MARA, Negeri Sembilan > Seremban Campus |
Programme: | Bachelor of Science (Hons.) (Mathematics) |
Keywords: | Electromyography, sEMG, ANN, muscle contractions |
Date: | 2024 |
URI: | https://ir.uitm.edu.my/id/eprint/95143 |
Download
![[thumbnail of 95143.pdf]](https://ir.uitm.edu.my/style/images/fileicons/text.png)
95143.pdf
Download (78kB)
Digital Copy
Physical Copy
ID Number
95143
Indexing
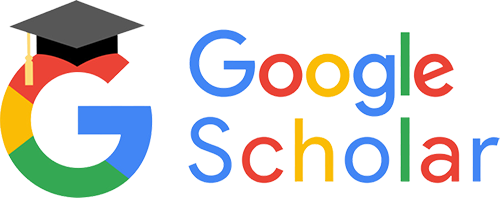
