Abstract
Agarwood oil is an essential oil which is a concentrated volatile aromatic compound that is produced by agarwood plant. It is widely being used as incense and fragrance in religious prayers and traditional ceremonies. The market price of agarwood oil being traded depends on its quality. It has been shown from literatures that agarwood oil quality can be classified into high or low quality. The latest method combined lab based GC-MS, z-score technique and ANN for agarwood oil quality classification, however the classification accuracy score achieved was only between range of 81- 86% and does not achieve 100%. This thesis describes a representation of k-nearest neighbors to classify the agarwood oil sample quality to high quality and low quality. In this study, the chemical compound of agarwood oil samples is obtained from GCMS analysis. Different type of feature scaling and data splitting technique were used in this experiment to analyze the effect on training the classifier model on the agarwood oil quality sample dataset. Using correlation-based feature selection, it was found that out of seven chemical compounds abundances only five were predictive. The k-nearest neighbors model was analyzed to get a comparison between different number of neighbors used to the overall classification accuracy and performance measure score of the model. The best scaling method found from the experiment is min-max scaling. From the results of the experiment, it shows that using stratified kfold cross validation splitting technique have a much better performance and stable model scores when compared to using hold-out test set technique. The k-NN classifier was built with number of neighbours ranging from 1 to 20 to obtain the best range of number of neighbours to be used to get the highest classification accuracy. The results from the experiment shows that the best range for number of neighbours used for k- NN classifier are 1 to 8 and best data splitting technique is stratified k-fold cross validation as the combination that have the highest classification accuracy to classify the quality of agarwood oil which is at 100%. The best number of neighbors parameters for the model is five.
Metadata
Item Type: | Thesis (Masters) |
---|---|
Creators: | Creators Email / ID Num. Abas, Mohamad Aqib Haqmi UNSPECIFIED |
Divisions: | Universiti Teknologi MARA, Shah Alam > Faculty of Electrical Engineering |
Programme: | Master of Science |
Keywords: | agar wood, plant, oil |
Date: | 2018 |
URI: | https://ir.uitm.edu.my/id/eprint/90061 |
Download
![[thumbnail of 90061.pdf]](https://ir.uitm.edu.my/style/images/fileicons/text.png)
90061.pdf
Download (1MB)
Digital Copy
Physical Copy

ID Number
90061
Indexing
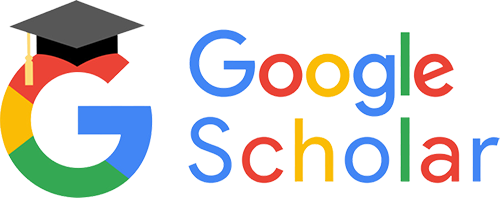
