Abstract
Customer churn is defined as the tendency of customers to cease doing business with a company in a given period. ABC Multistate Bank faces the challenges to hold clients. The purpose of this study is to apply machine learning algorithms to develop the most effective model for predicting bank customer churn. In this study, six supervised machine learning methods, K-Nearest Neighbors, Support Vector Machine, Naïve Bayes, Decision Tree, Random Forest, and Extreme Gradient Boosting (XGBoost), are applied to the churn prediction model using Bank Customer Data of ABC Multistate Bank obtained from Kaggle. The results showed that XGBoost outperformed the other six classifiers, with an accuracy rate of 84.76%, an F1 score of 56.95%, and a ROC curve graph of 71.64%. The bank may use XGBoost model to accurately identify customers who are at risk of leaving, concentrate their efforts on them, and possibly make a profit. Future research should focus on various machine learning approaches for determining the most accurate models for bank customer churn datasets.
Metadata
Item Type: | Article |
---|---|
Creators: | Creators Email / ID Num. Hui, Shan Hon honhuishan@gmail.com Khai, Wah Khaw khaiwah@usm.my XinYing, Chew xinying@usm.my Wai, Peng Wong waipeng.wong@monash.edu |
Subjects: | Q Science > Q Science (General) > Machine learning |
Divisions: | Universiti Teknologi MARA, Shah Alam > Faculty of Computer and Mathematical Sciences |
Journal or Publication Title: | Malaysian Journal of Computing (MJoC) |
UiTM Journal Collections: | UiTM Journals > Malaysian Journal of Computing (MJoC) |
ISSN: | 2600-8238 |
Volume: | 8 |
Number: | 2 |
Page Range: | pp. 1602-1619 |
Keywords: | Bank Customer Churn, Machine Learning, Supervised Machine Learning |
Date: | October 2023 |
URI: | https://ir.uitm.edu.my/id/eprint/86389 |
Download
![[thumbnail of 86389.pdf]](https://ir.uitm.edu.my/style/images/fileicons/text.png)
86389.pdf
Download (633kB)
ID Number
86389
Indexing
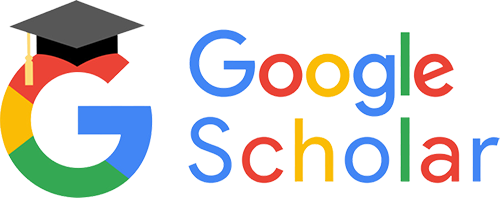
