Abstract
This report is about the Artificial Neural Networks (ANN) are used to predict incipient faults in power transformers oil. The prediction is performed through the Dissolved Gas Analysis (DGA) method. The function of this method is for detect and diagnose the different types of incipient faults that occur in power trasformers. By interpretation of dissolved gasses in oil insulation of power transformers, this method was applied in the Artificial Neural Networks (ANN) to classify the different faults by using the DGA method. In DGA method, the Roger's Ratio and International Electrotechnical Commission (IEC) Ratio were applied into ANN to see the performance of ANN's network. For assessment, two set databases are employed: Roger's ratio and IEC ratio. The data bases are collected from Tenaga Nasional Berhad (TNB) data. The results show these methods were used to predicting the fault more than 90% of accuracy m best case.
Metadata
Item Type: | Thesis (Degree) |
---|---|
Creators: | Creators Email / ID Num. Mansor, Nur Diyana UNSPECIFIED |
Contributors: | Contribution Name Email / ID Num. Thesis advisor Johari, Dalina UNSPECIFIED |
Subjects: | Q Science > QA Mathematics > Instruments and machines > Electronic Computers. Computer Science > Neural networks (Computer science) T Technology > TK Electrical engineering. Electronics. Nuclear engineering > Electronics |
Divisions: | Universiti Teknologi MARA, Shah Alam > Faculty of Electrical Engineering |
Programme: | Bachelor of Engineering (Hons.) Electrical |
Related URLs: | |
Keywords: | Artificial Neural Networks, incipient faults, power transformers oil |
Date: | 2013 |
URI: | https://ir.uitm.edu.my/id/eprint/84633 |
Download
![[thumbnail of 84633.pdf]](https://ir.uitm.edu.my/style/images/fileicons/text.png)
84633.pdf
Download (1MB)
Digital Copy
Physical Copy

ID Number
84633
Indexing
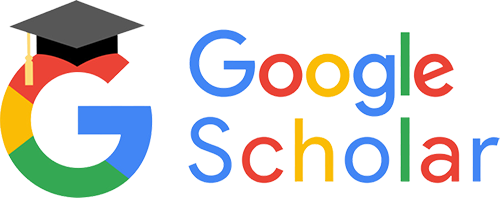
