Abstract
Brain-Computer Interface (BCI) is a new emerging technology that provides an alternative medium of communication where the human brain (via electroencephalography signals) can communicate with the computer and other electronic peripherals. In this particular domain, various studies have been conducted to analyse and decode Electroencephalography (EEG) signals. This study aims to identify and adopt suitable methods and analyses to distinguish distinct brainwave patterns with the occurrence of actual and imaginary hand lifting movements. Another aim of this study is to find. a correlation between actual and imaginary EEG triggered data, specifically on lifting left and right hand. Scoping down into a more specific area, this research focuses on using different types of feature extraction methods by experimenting with Artificial Neural Network (ANN) and Support Vector Machine (SVM) for classification. While correlation analysis was performed to find the relationship between different events. For data collection purpose, an experimental procedure is designed especially to·record.EEG signals from the subjects while doing either the actual or imaginary hand movements. The recorded raw EEG signals were first preprocessed before they can be analysed. Coefficient values obtained from the extracted features i.e. Energy Spectral Density (ESD) and Power Spectral Density (PSD) were fed as inputs to the ANN and SVM classifier for classification purpose. For SVM classifier the analysis is divided into three types of kernel function i.e. Linear Kernel, Quadratic Kernel and Polynomial Kernel. Based on the obtained results, it is shown that the extracted PSD features can work well with ANN and SVM classifier. The average accuracy to differentiate between actual movements of lifting the left and right hand was obtained at 93% when using ANN classifier, while in average the accuracy measure obtained for SVM classifier was more than 70%. The average· accuracy for differentiating between imaginary lifting left and right hand was acquired at 76.25% by the ANN classifier and, 77.5% by the SVM classifier. While for the combined dataset of both actual and imaginary, the average accuracy values were 85.65% by the ANN classifier and 82.9% by the SVM classifier.
Metadata
Item Type: | Thesis (Masters) |
---|---|
Creators: | Creators Email / ID Num. Hamzah, Nabilah UNSPECIFIED |
Contributors: | Contribution Name Email / ID Num. Thesis advisor Mohamad Zaini, Norliza UNSPECIFIED |
Subjects: | Q Science > QA Mathematics > Instruments and machines > Electronic Computers. Computer Science > Neural networks (Computer science) |
Divisions: | Universiti Teknologi MARA, Shah Alam > Faculty of Electrical Engineering |
Programme: | Master of Science (Electrical Engineering) |
Keywords: | Electroencephalography, brainwave patterns, Artificial Neural Network |
Date: | 2018 |
URI: | https://ir.uitm.edu.my/id/eprint/84289 |
Download
![[thumbnail of 84289.pdf]](https://ir.uitm.edu.my/style/images/fileicons/text.png)
84289.pdf
Download (405kB)
Digital Copy
Physical Copy

ID Number
84289
Indexing
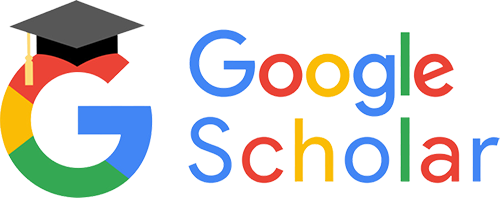
