Abstract
Malware is one of the most harmful forms of attack on computers because of its passive approach and hidden execution. The most widespread type of malicious software that discreetly monitors user activities and logs keystrokes is called keylogging malware. Accordingly, the goal of this study are to create a detection model based on both supervised machine learning on keylogger dataset. Plus, to analyse the efficiency of a detection model on keylogger dataset by evaluating a selection of attributes. Besides, to test the accuracy of detection models on keylogger dataset comparing two machine learning algorithms. This study is carried out through the utilisation of two machine learning techniques, namely Decision Tree and Naive Bayes, on Jupyter Notebook in order to conduct an analysis of the Keylogger Detection dataset obtained from a trustworthy website known as Kaggle. There are a few outcomes that have been achieved to decide between those two machine learning methods that have better accuracy to carry out analysis on the dataset which of the two, but rather Decision Tree, have the greater accuracy. Early identification of a keylogger malware attack could prevent hackers from accessing personal user data and reduce the likelihood of infiltration, which could reveal account information, credit cards, usernames, passwords, and other data. In this way, we can decrease the likelihood of being the victim of a spyware attack and losing our information. It is intended that this initiative would deliver benefits to all of the users and be useful to them.
Metadata
Item Type: | Student Project |
---|---|
Creators: | Creators Email / ID Num. Abdul Rahman, Muhammad Faiz Hazim UNSPECIFIED |
Contributors: | Contribution Name Email / ID Num. Advisor Hj Mat Taib, Abidah UNSPECIFIED |
Subjects: | T Technology > TK Electrical engineering. Electronics. Nuclear engineering > Telecommunication > Computer networks. General works. Traffic monitoring > Intrusion detection systems (Computer security). Computer network security. Hackers |
Divisions: | Universiti Teknologi MARA, Perlis > Arau Campus > Faculty of Computer and Mathematical Sciences |
Programme: | Bachelor of Computer Science (Hons.) Data Communication and Networking |
Keywords: | Malware, Keylogger detection, machine learning |
Date: | 2022 |
URI: | https://ir.uitm.edu.my/id/eprint/83337 |
Download
![[thumbnail of 83337.pdf]](https://ir.uitm.edu.my/style/images/fileicons/text.png)
83337.pdf
Download (157kB)
Digital Copy
Physical Copy

ID Number
83337
Indexing
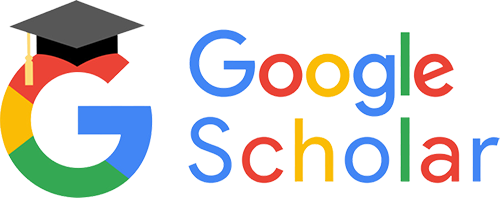
