Abstract
Because of various security concerns and cyberattacks, cybersecurity is crucial in today's environment. In addition, malware has evolved quickly in recent years. Machine learning is utilised for malware detection with the advancement of malware analysis. The comparison of malware detection model utilising supervised machine learning techniques is the main goal of this project. The objective of this project is to develop the Windows malware detection model using supervised machine learning in Decision Tree, K-NN and Naïve Bayes, to evaluate the performance of malware detection in term of testing and training of the features selection and to compare the accuracy detection model in all three machine learning algorithms. The Windows malware dataset has been trained and tested by these three machine learning algorithms to get the percentage detection accuracy. Then, the outcomes demonstrated that the best classifier for categorizing our data with 0.96% accuracy is the Decision Tree machine learning algorithm. When comparing the accuracy of a malware detection model, it is excellent if there are numerous machine learning algorithms and more malware datasets included.
Metadata
Item Type: | Student Project |
---|---|
Creators: | Creators Email / ID Num. Mohd Shahirudin, Syamir UNSPECIFIED |
Contributors: | Contribution Name Email / ID Num. Advisor Mohd Fuzi, Mohd Faris UNSPECIFIED |
Subjects: | T Technology > TK Electrical engineering. Electronics. Nuclear engineering > Telecommunication > Computer networks. General works. Traffic monitoring > Intrusion detection systems (Computer security). Computer network security. Hackers |
Divisions: | Universiti Teknologi MARA, Perlis > Arau Campus > Faculty of Computer and Mathematical Sciences |
Programme: | Bachelor of Science (Hons.) Data Communication and Networking |
Keywords: | cybersecurity, malware, Machine learning |
Date: | 2022 |
URI: | https://ir.uitm.edu.my/id/eprint/83326 |
Download
![[thumbnail of 83326.pdf]](https://ir.uitm.edu.my/style/images/fileicons/text.png)
83326.pdf
Download (160kB)
Digital Copy
Physical Copy

ID Number
83326
Indexing
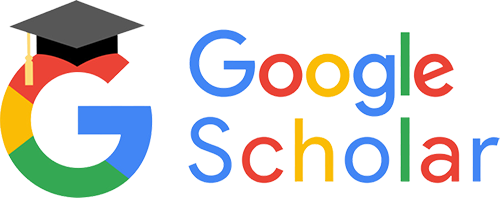
