Abstract
Valuers face various challenges in determining property prices and rental values due to their heavy dependence on market data. The use of existing databases in property valuation assignments presents intrinsic challenges where the valuer might derive incorrect assumptions in analysing value-issued comparable data. It is worth noting that when predicting property values and rentals, appraisers and investors cannot rely on historical market data from real estate transactions. With the increasing spectrum of Industrial Revolution 4.0, the introduction of certain computing techniques optimised the advancements in data science technologies are unavoidably the best options. Thus, this research aims to develop the office building rental prediction model based on machine learning. To fulfil this aim, this research proposed three (3) objectives, firstly to identify the factors affecting office building rental based on the statistics from previous empirical study through the systematic literature review.
Metadata
Item Type: | Thesis (Masters) |
---|---|
Creators: | Creators Email / ID Num. Abdul Salam, Muhamad Harussani 2021927371 |
Contributors: | Contribution Name Email / ID Num. Thesis advisor Mohd, Thuraiya UNSPECIFIED |
Subjects: | H Social Sciences > HD Industries. Land use. Labor > Service industries |
Divisions: | Universiti Teknologi MARA, Shah Alam > College of Built Environment |
Programme: | Master of Science (Built Environment) – AP781 |
Keywords: | Office Building, Rental, Prediction, Machine Learning |
Date: | 2022 |
URI: | https://ir.uitm.edu.my/id/eprint/82868 |
Download
![[thumbnail of 82868.pdf]](https://ir.uitm.edu.my/style/images/fileicons/text.png)
82868.pdf
Download (181kB)
Digital Copy
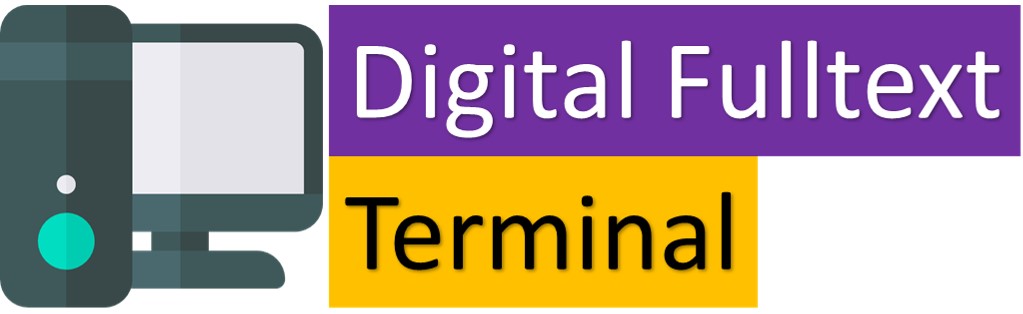
Physical Copy
ID Number
82868
Indexing
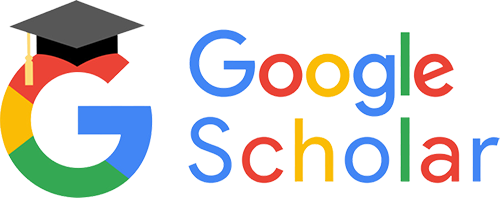
