Abstract
Congestion problem is a crucial issue in power system. Its occurrence is closely related to loss increment and voltage decay in power system. The increment of load in a transmission system is one of the main factors that causes current increase. This leads to loss increment, while at the same time affecting the congestion event in the system. The impact leads to the increment in generation cost during congestion. Therefore, congestion management needs to be performed properly in order to deliver enough power to the system resulted by transmission line congestion. Failure to handle this situation may lead to bigger problems such as voltage collapse and cascading blackout. This thesis presents computational intelligence-based technique for congestion management and compensation scheme in power systems. In this study, a new model termed as Integrated Multilayer Artificial Neural Networks (IMLANNs) is developed to predict congested line and voltage stability index separately. Consequently, a new optimization technique termed as Clonal Evolutionary Particle Swarm Optimization (CEPSO) was developed. CEPSO integrates the element of cloning and swarm in the original Evolutionary Programming algorithm. CEPSO is initially used to optimize the location and sizing of FACTS devices for compensation scheme. In this study, Static VAR Compensator (SVC) and Thyristor Control Static Compensator (TCSC) are the two chosen Flexible AC Transmission System (FACTS) devices used in this compensation scheme.
Metadata
Item Type: | Thesis (PhD) |
---|---|
Creators: | Creators Email / ID Num. Mohd Ali, Nur Zahirah 2013231252 |
Contributors: | Contribution Name Email / ID Num. Thesis advisor Musirin, Ismail UNSPECIFIED |
Subjects: | T Technology > TK Electrical engineering. Electronics. Nuclear engineering |
Divisions: | Universiti Teknologi MARA, Shah Alam > Faculty of Electrical Engineering |
Programme: | Doctor of Philosophy (Electrical Engineering) – EE950 |
Keywords: | Congestion, compensation scheme, power system |
Date: | 2019 |
URI: | https://ir.uitm.edu.my/id/eprint/82783 |
Download
![[thumbnail of 82783.pdf]](https://ir.uitm.edu.my/style/images/fileicons/text.png)
82783.pdf
Download (156kB)
Digital Copy
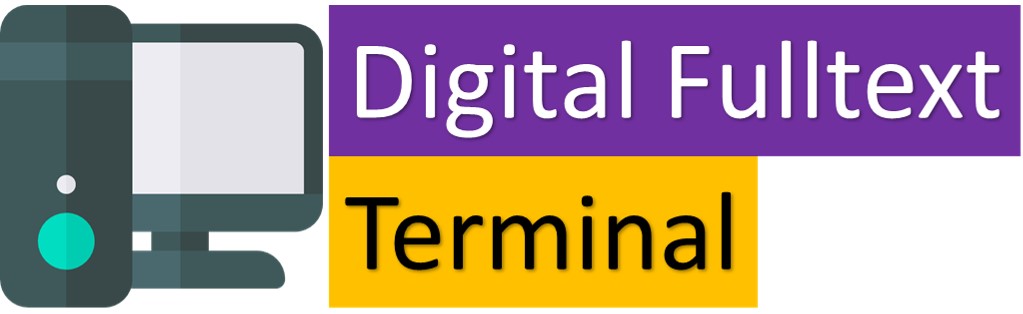

Physical Copy
ID Number
82783
Indexing
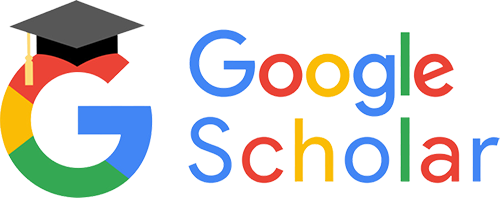
