Abstract
The Global Navigation Satellite System (GNSS) is subjected to various noise sources affecting positioning accuracy during real-time implementation. The development of Real Time Kinematics (RTK) and GNSS was introduced to enhance positioning accuracy. However, the RTK-GNSS is not able to achieve a fixed integer (centimetre) solution most of the time during dynamic applications. In recent years, the Gaussian Process (GP) has been employed to model GNSS measurement errors during dynamic applications. GP is a time-consuming process and computationally expensive, having a possible tendency to produce the best fit model. Moreover, the performance of GP of model fit on trajectory data has not been studied. Hence, the three main objectives of this work are: (i) to perform data logging and analyse the characteristics of RTK-GNSS errors; (ii) to model localization errors of RTK-GNSS dynamic measurements using sparse Gaussian process regression (GPR), which offers less computational complexity and (iii) optimize the performance of the sparse GPR model. The RTK-GNSS used in this work comprised two EMLID Reach RS. Data logging during dynamic applications was conducted on two trajectories under multipath and one without multipath when operating under short baseline and clear sky conditions. Seven sets of data were collected for each trajectory. The collected data was analyzed based on two-dimension (2D) positioning accuracy and availability; probabilistic distribution of the error residuals; autocorrelation function (ACF) and partial correlation function (PACF) to evaluate correlations in the data. The analysis revealed the presence of correlated noise in the measurements which did not conform consistently to any probabilistic distribution. Subsequently, sparse GPR models were developed for each trajectory based on five types of kernel functions. The best performing kernel function was chosen to implement sparse GPR that could predict the x and y axes coordinates for each trajectory. Next, Linear Regression-Autoregressive Integrated Moving Average (LR-ARIMA) error models were also developed to improve the positioning errors in the respective axes. The LR-ARIMA models were estimated based on the ACF and PACF relations and the Akaike Information Criterion (AIC). The developed LR-ARIMA models were also used to optimize the sparse GPR models by combining the GP models with LR-ARIMA (GPR-LR-ARIMA). The performance of the GPR, LR-ARIMA, and GPR-LR-ARIMA models, respectively, were evaluated and compared in terms of Mean Absolute Error (MAE) and Root Mean Square Error (RMSE) on 2D position errors. The models’ residuals were analyzed using histogram, ACF, PACF and Quantile-Quantile Plot (QQ-Plot) to evaluate model fitting. The MAE of 2D position errors has been improved by up to 60.38% using sparse GPR models, up to 51.94% when using the LR-ARIMA models and 84.73% using the GPR-LR-ARIMA models when compared to the original logged data. In conclusion, an integration of the GPR and LR-ARIMA was feasible to improve positioning errors with better model fit.
Metadata
Item Type: | Thesis (Masters) |
---|---|
Creators: | Creators Email / ID Num. Nahar, Ravenny Sandin 2019679846 |
Contributors: | Contribution Name Email / ID Num. Thesis advisor Ng, Kok Mun UNSPECIFIED |
Subjects: | Q Science > Q Science (General) > Back propagation (Artificial intelligence) T Technology > T Technology (General) |
Divisions: | Universiti Teknologi MARA, Shah Alam > College of Engineering |
Programme: | Master of Science (Electrical Engineering) – EE750 |
Keywords: | GNSS, GPR, GP |
Date: | 2023 |
URI: | https://ir.uitm.edu.my/id/eprint/82282 |
Download
![[thumbnail of 82282.pdf]](https://ir.uitm.edu.my/style/images/fileicons/text.png)
82282.pdf
Download (235kB)
Digital Copy
Physical Copy
ID Number
82282
Indexing
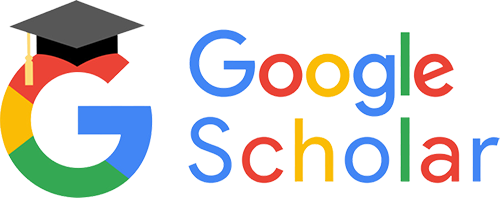
