Abstract
The current practice of mobile network management involves human interaction and human work ranging from conducting drive tests that can evaluate mobile network performance and coverage to identify customer complaints. Conducting drive test causing extended human work and time-consuming when processing a large and complex data. Predictive network analytics related to network management involves predicting network performance at locations or times in which no direct measurement data is available or solving missing values in the data collection when conducting the drive test. One of the major challenges when applying machine learning is to identify the best algorithm from a variety of algorithms to solve a problem. This study aims to propose the best machine learning algorithm for predicting mobile network performance. This can be achieved by comparing several types of machine learning algorithms in predicting mobile network performance. The methodology includes drive test measurement for data collection, exploratory data analysis, data preparation, and applying machine learning algorithms to predict mobile network performance. Since throughput feature has a strong correlation with the signal strength performance, it is the targeted parameter in the network performance prediction.
Metadata
Item Type: | Thesis (Masters) |
---|---|
Creators: | Creators Email / ID Num. Wahid, Muhammad Hazim 2021186713 |
Contributors: | Contribution Name Email / ID Num. Thesis advisor Abdul Razak, Nur Idora UNSPECIFIED |
Subjects: | Q Science > Q Science (General) > Cybernetics T Technology > TK Electrical engineering. Electronics. Nuclear engineering > Telecommunication > Wireless communication systems. Mobile communication systems. Access control |
Divisions: | Universiti Teknologi MARA, Shah Alam > College of Engineering |
Programme: | Master of Science (Electrical and Electronic Communication) |
Keywords: | Mobile network management, human interaction, human work |
Date: | 2022 |
URI: | https://ir.uitm.edu.my/id/eprint/75721 |
Download
![[thumbnail of 75721.pdf]](https://ir.uitm.edu.my/style/images/fileicons/text.png)
75721.pdf
Download (145kB)
Digital Copy
Physical Copy
ID Number
75721
Indexing
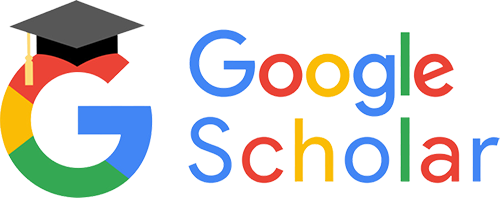
