Abstract
Alzheimer's disease (AD) is a neurodegenerative disorder that can be characterised by the gradual progression of memory loss, impairment of cognitive function, and progressive disability. Currently, there are no treatments available for AD. Detection of biomarkers would assist in early prediction of AD. Prevalent technologies of high throughput in genomics have opened a new horizon to achieve this purpose. Transcriptomics, metabolomics, and cytokinomics data are among the multi-omics data used in modern genomics studies. Multi-omics data is high dimensional data where the number of dimensions is larger than the number of sample observations. Additionally, due to the large number of features, there are issues of multicollinearity and complete separation. Therefore, questions have been raised about how to analyse multi-omics high dimensional data and what the best classifiers for the classification of AD are.
Metadata
Item Type: | Thesis (PhD) |
---|---|
Creators: | Creators Email / ID Num. Abdullah, Mohammad Nasir UNSPECIFIED |
Contributors: | Contribution Name Email / ID Num. Thesis advisor Yap, Bee Wah UNSPECIFIED |
Subjects: | R Medicine > RC Internal Medicine > Neuroscience. Biological psychiatry. Neuropsychiatry > Psychiatry > Psychoses > Dementia > Alzheimer's disease |
Divisions: | Universiti Teknologi MARA, Shah Alam > Faculty of Computer and Mathematical Sciences |
Programme: | Doctor of Philosophy (Statistics) - CS953 |
Keywords: | Alzheimer's, blood, neurodegenerative |
Date: | 2022 |
URI: | https://ir.uitm.edu.my/id/eprint/75599 |
Download
![[thumbnail of 75599.pdf]](https://ir.uitm.edu.my/style/images/fileicons/text.png)
75599.pdf
Download (289kB)
Digital Copy
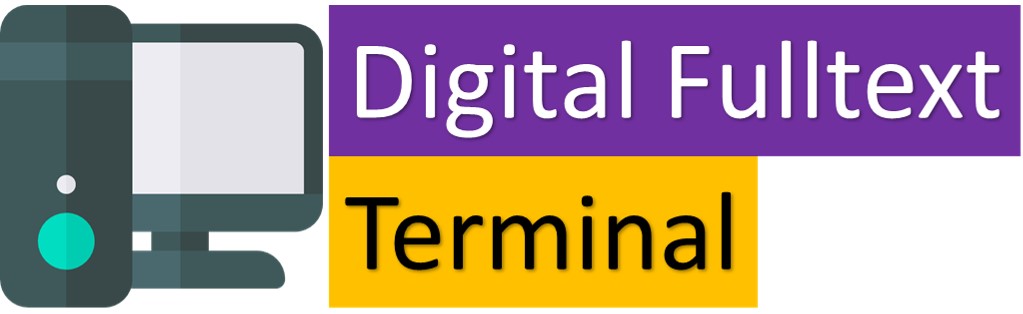

Physical Copy
ID Number
75599
Indexing
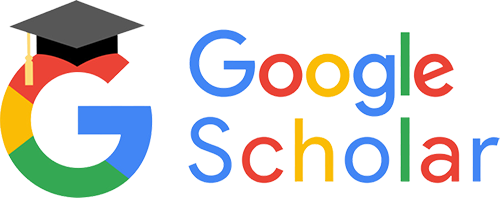
