Abstract
Agarwood oil is a highly traded essential oil with a high price tag. The oil is extracted from the stems of resin-impregnated agarwood trees. Agarwood essential oil has been used in various applications, including incense, perfumery, medicinal purposes and religious ceremonies. Conventionally, agarwood essential oil is graded manually by a human expert sensory panel based on its physical properties such as odor, color intensity and infection level. The method for obtaining the quality of agarwood oil is time consuming due to the large number of test samples. Furthermore, humans are prone to fatigue as a result of continuously handling the samples. This research proposes an intelligent technique for grading agarwood essential oil based on its chemical properties using the artificial neural network (ANN) technique. Initially, the sample data of chemical compounds of selected agarwood oil were measured using gas chromatography-mass spectrometry (GC-MS) and then pre-processed in sequence by three pre-processing techniques, namely Z-score, PCA and stepwise regression; the number of chemical compounds was reduced from more than ninety to only seven, three and four, respectively. These significant chemical compounds from each preprocessing technique, were used as the input to ANN. To classify the agarwood essential oil into high and low qualities, this research applied a three backpropagation training algorithms—scaled conjugate gradient (SCG), Levenberg Marquardt (LM) and resilient backpropagation (RBP). The training and validation of the ANN was based on optimization of its training parameters and guided by the convergence of the mean squared errors (MSE). The result revealed that the ANN performance had the highest accuracy when training using the LM training algorithm. The ANN with the seven inputs (seven significant compounds from Z-score pre-processing technique) trained by one hidden neuron of LM algorithm provided the best performance with 100% for accuracy, specificity, sensitivity and precision as well as minimum convergence epoch.
Metadata
Item Type: | Thesis (PhD) |
---|---|
Creators: | Creators Email / ID Num. Mahabob, Noratikah Zawani 2020909721 |
Contributors: | Contribution Name Email / ID Num. Thesis advisor Mohd Yusoff, Zakiah UNSPECIFIED |
Subjects: | R Medicine > RM Therapeutics. Pharmacology > Drugs and their actions > Aromatic plants. Aromatherapy. Including essences and essential oils |
Divisions: | Universiti Teknologi MARA, Shah Alam > College of Engineering |
Programme: | EE950 |
Keywords: | Agarwood oil, artificial neural network, intelligent grading |
Date: | 2022 |
URI: | https://ir.uitm.edu.my/id/eprint/75214 |
Download
![[thumbnail of 75214.pdf]](https://ir.uitm.edu.my/style/images/fileicons/text.png)
75214.pdf
Download (128kB)
Digital Copy
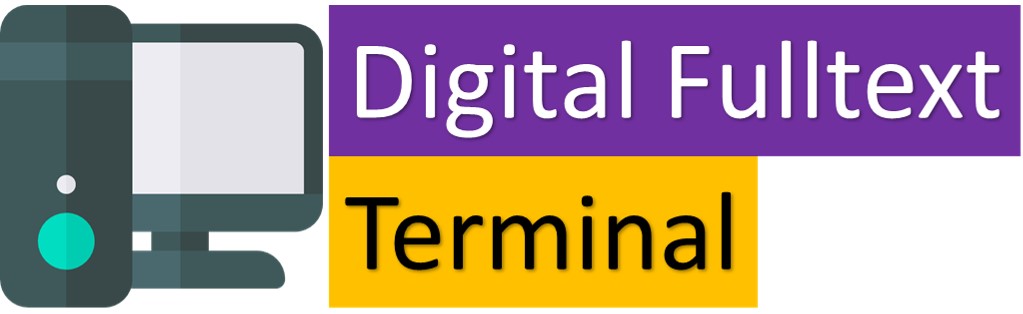

Physical Copy
ID Number
75214
Indexing
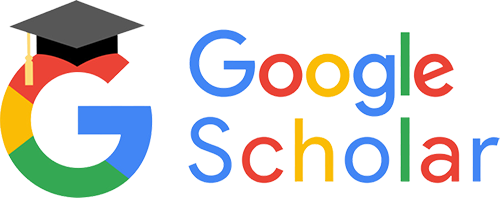
