Abstract
The assessment of parameter estimation and model fit are important in Structural
Equation Modelling (SEM). Several goodness-of-fit (GoF) measures are affected by
sample size and the number of parameters to be estimated. In SEM, a larger sample
size is required to test a complex model involving large number of parameters to be
estimated. One of the solutions is to reduce the number of parameters to be estimated
in a given structural model by considering item-parcelling (IP). Although the use of
IP is widely applied in research studies, parcelling of items still remain a controversial
issue. The IP technique makes multidimensional constructs seem like unidimensional
and may cause loss of information and the meaning of the latent construct. There is a
need to examine and compare the item-level (IL) and IP models to determine if IP has
a profound effect on the structural model parameter estimates and model fit measures.
Previous research highlighted that the number of items for parcel and the method to
create parcels are the main problematic issues in IP. There are many possible ways to
allocate items to parcels and different ways of IP will generate different results for the
structural model. Thus, this study determined the effects of IP based on different
number of parcels on parameter estimates and model fit for normal and non-normal
data in SEM. The data were simulated for IL and IP (2I6P, 3I4P, 4I3P, and 6I2P)
models. Then, the effectiveness of existing item-parcelling (random, correlation, and
single-factor) and the proposed cluster-parcelling (IPCM) methods were determined
based on the performance of parameter estimates and model fit for normal and nonnormal
data in SEM. The data were generated for nine different sample sizes (100,
150, 200, 250, 300, 500, 1000, 1500, and 2000) and three different data distributions
(normal, moderately non-normal, and severely non-normal) for each simulation phase.
Finally, the effect of proposed IPCM method on parameter estimates and model fit
were examined for a real-dataset. The simulation results indicated that model fit
improved with implementation of IP and 2I6P model have the least biased parameter
estimates among the IP methods. Under normal and moderate non-normal
distributions, across all sample sizes, the bias of parameter estimates is acceptable for
all IP methods except correlation method. Meanwhile, the parameter estimates are less
bias for random, single-factor, and IPCM methods for large sample sizes (n≥500).
Under severe non-normal distribution, the bias of parameter estimates is acceptable
for random, single-factor and IPCM methods when sample sizes were 150 and above,
while the parameter estimates are less bias for random and IPCM methods when
sample size is very large (n≥1000). Based on goodness-of-fit index (GFI), adjusted
GFI (AGFI), and root mean square error of approximation (RMSEA), IPCM method
produced the best model fit for IP models. Meanwhile, based on normed fit index
(NFI), Tucker-Lewis index (TLI), and comparative fit index (CFI), the model fit is
better for single and IPCM methods compared to others. The results indicated that the
proposed IPCM method can be considered in application of IP in SEM since the
parameter estimates for IPCM method is the closest to the IL model and the model fit
based on GFI, AGFI, NFI, TLI, and CFI improved with implementation of IP.
Metadata
Item Type: | Thesis (PhD) |
---|---|
Creators: | Creators Email / ID Num. Kamaruddin, Ainur Amira 2013305787 |
Contributors: | Contribution Name Email / ID Num. Thesis advisor Yap, Bee Wah UNSPECIFIED Thesis advisor Mohd Deni, Sayang UNSPECIFIED |
Subjects: | Q Science > QA Mathematics > Mathematical statistics. Probabilities Q Science > QA Mathematics > Multivariate analysis. Cluster analysis. Longitudinal method > Path analysis. Structural equation modeling |
Divisions: | Universiti Teknologi MARA, Shah Alam > Faculty of Computer and Mathematical Sciences |
Programme: | Doctor of Philosophy (Statistics) |
Keywords: | Structural equation modelling (SEM), clustering techniques, distance metrics |
Date: | 2022 |
URI: | https://ir.uitm.edu.my/id/eprint/66951 |
Download
![[thumbnail of 66951.pdf]](https://ir.uitm.edu.my/style/images/fileicons/text.png)
66951.pdf
Download (255kB)
Digital Copy
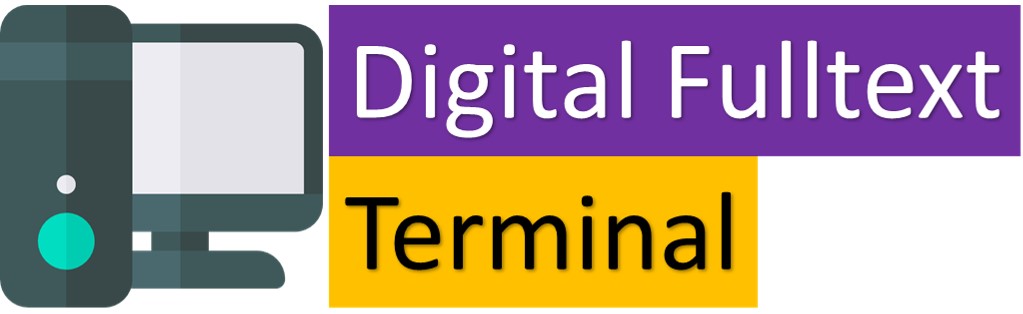
Physical Copy
ID Number
66951
Indexing
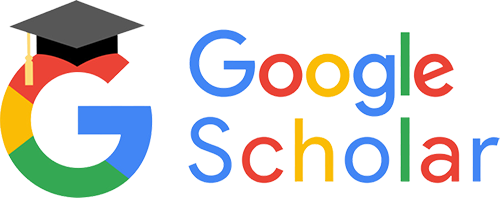
