Abstract
This thesis presents a simulation study on parameter estimation for binary and multinomial logistic regression, and the extension of the clustering partitioning strategy for goodness-of-fit test to multinomial logistic regression model. The motivation behind this study is influenced by two main factors. Firstly, parameter estimation is often sensitive to sample size and types of data. Simulation studies are useful to assess and confirm the effects of parameter estimation for binary and multinomial logistic regression under various conditions. The first phase of this study covers the effect of different types of covariate, distributions and sample size on parameter estimation for binary and multinomial logistic regression model. Data were simulated for different sample sizes, types of covariate (continuous, count, categorical) arid distributions (normal or skewed for continuous variable). The simulation results show that the effect of skewed and categorical covariate reduces as sample size increases. The parameter estimates for normal distribution covariate apparently are less affected by sample size. For multinomial logistic regression model with a single covariate, a sample size of at least 300 is required to obtain unbiased estimates when the covariate is positively skewed or is a categorical covariate. A much larger sample size is required when covariates are negatively skewed. In Phase 2, we investigate the goodness-of-fit (GoF) tests for multinomial logistic regression. Goodness-of-fit tests are important to assess if the model fits the data. We investigated the Type I error and power of two goodness-of-fit tests for multinomial logistic regression via a simulation study. The GoF test using partitioning strategy (clustering) in the covariate space, XP*G w a s compared with another test, Cg which was based on grouping of predicted probabilities. The power of both tests was investigated when quadratic term or interaction term were omitted from the model. The proposed test XP*G shows good Type I error and ample power except for multinomial models with highly skewed covariate distribution. Additionally, the proposed test XP*G has good power in detecting omission of continuous interaction term. Further simulation results showd that partitioning strategy using Hierarchical Clustering with Canberra distance, %C,G performs better than XP*G (Hiearchical clustering with Euclidean distance) and XI*G (Partitioning using k-medoids). The application on a real dataset confirmed the simulation results. The simulation and analyses were carried out using R, an open-source programming language for statistical computing and graphics.
Metadata
Item Type: | Thesis (PhD) |
---|---|
Creators: | Creators Email / ID Num. Abdul Hamid, Hamzah 2013641848 |
Contributors: | Contribution Name Email / ID Num. Thesis advisor Yap, Bee Wah UNSPECIFIED |
Subjects: | Q Science > QA Mathematics > Multivariate analysis. Cluster analysis. Longitudinal method > Regression analysis. Correlation analysis. Spatial analysis (Statistics) |
Divisions: | Universiti Teknologi MARA, Shah Alam > Faculty of Computer and Mathematical Sciences |
Programme: | Doctor of Philosophy |
Keywords: | General linear model, logistic regression model, programming language |
Date: | 2017 |
URI: | https://ir.uitm.edu.my/id/eprint/66514 |
Download
![[thumbnail of 66514.pdf]](https://ir.uitm.edu.my/style/images/fileicons/text.png)
66514.pdf
Download (180kB)
Digital Copy
Physical Copy

ID Number
66514
Indexing
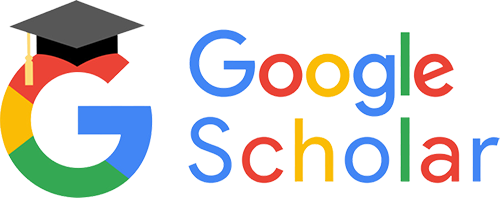
