Abstract
This study uses k-Nearest Neighbour (k-NN) in segmentation of brain abnormality. Segmentation is a process of partitioning a digital image into multiple regions or set of pixels. The goal of segmentation is to simplify and/or change the representation of an image into something that is more meaningful and easier to analyze. Segmentation of MRI image is an important part of brain imaging research. In this study, k-NN segmentation uses 150 images as testing data to test this prototype. These data are designed by cutting various shapes and size of various abnormalities and pasting it onto normal brain size which are has various category of background such as low, medium and high background gray level value. The experimental results show the good segmentation for medium and low background grey level value for light abnormality. Dark abnormality for each type of background also produced good segmentation. However, high background gray level value for light abnormality produced poor segmentation because texture of background and light abnormality seen like same. In the future, this project needs to use other techniques to produce accuracy result for light and dark abnormality even k-NN segmentation produced good segmentation.
Metadata
Item Type: | Thesis (Degree) |
---|---|
Creators: | Creators Email / ID Num. Megat Mohd Haniff, Puteri Nurain 2008717121 |
Contributors: | Contribution Name Email / ID Num. Thesis advisor Abd. Khalid, Noor Elaiza UNSPECIFIED |
Divisions: | Universiti Teknologi MARA, Shah Alam > Faculty of Computer and Mathematical Sciences |
Programme: | Degree of Bachelor of Science |
Keywords: | K-NN, brain, abnormality |
Date: | 2010 |
URI: | https://ir.uitm.edu.my/id/eprint/63667 |
Download
![[thumbnail of 63667.pdf]](https://ir.uitm.edu.my/style/images/fileicons/text.png)
63667.pdf
Download (395kB)
Digital Copy
Physical Copy

ID Number
63667
Indexing
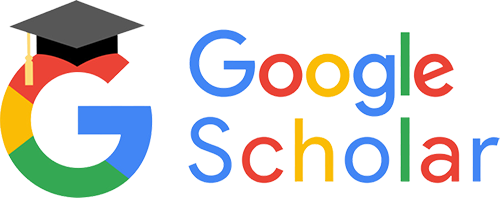
