Abstract
Asphalt pavement cracks are one of the major road damage problems in the civil field as they may potentially threaten road and highway safety. Crack detection and classification are challenging tasks due to the complicated pavement conditions such as the presence of shadows, oil stains, and water spots. These conditions will eventually result in poor visual and low contrast between cracks and the surrounding pavement. Therefore, in tackling these issues, this study proposes a fully automated pavement crack detection and classification using a new Deep Convolutional Neural Network architecture (DCNN) called hierarchical DCNN (Hi-DCNN). The image of pavement cracks is manually prepared in RGB format with a dimension of 1024×768 pixels. This image is captured using NIKON digital camera and segmented into patches (32×32 pixels) as a training dataset from the original pavement cracks. To ease these processes, this study introduces a new app, CrackLabel, that can automatically label patches in the crack images into two groups, crack and non-crack. The CrackLabel utilises a special design image thresholding algorithm known as Global and Lower Quartile Average Intensity (GLQAI). In the first part, a DCNN structure, DCNN-1, is proposed to perform crack detection based on the labelled patches as its input. This is necessary to build training and testing datasets for the network. As a result, the network managed to achieve better performance in crack detection with 98.50%, 98.85%, 98.68%, and 98.67% for recall, precision, accuracy, and F-score respectively. As for the second part involving the crack classification process, DCNN-2 is proposed to classify the detected crack images, obtained from the previous step. This stage is performed to classify images into three groups which are transverse, longitudinal, and alligator cracks. The Hi-DCNN structure achieved promising accuracy of 96.80%, 97.41%, and 95.58% for transverse, longitudinal and alligator respectively. The last part is the integration of the optimized performance networks from the Hi-DCNN, which proposes a new app system that can automatically detect and classify pavement cracks. This app can facilitate and assist civil surveyors in determining pavement distress through a simple and user-friendly interface. For future work, this study suggests a real-time automated app that allows the integration between crack images and a manual operated digital camera to facilitate the automated process. In addition, the conversion of the RGB images into HSV colour space and further evaluation on the crack depth analysis help to obtain fine-detailed information for handling network robustness.
Metadata
Item Type: | Thesis (PhD) |
---|---|
Creators: | Creators Email / ID Num. Muhamed Yusof, Nor Aizam 2017693682 |
Contributors: | Contribution Name Email / ID Num. Thesis advisor Osman, Muhammad Khusairi (Dr.) UNSPECIFIED |
Subjects: | Q Science > QA Mathematics > Instruments and machines > Electronic Computers. Computer Science > Neural networks (Computer science) T Technology > TE Highway engineering. Roads and pavements > Pavements and paved roads |
Divisions: | Universiti Teknologi MARA, Shah Alam > College of Engineering |
Programme: | Doctor of Philosophy (Electrical Engineering) |
Keywords: | Pavement distress; inspection; pavement crack detection; deep neural network; image labeling |
Date: | August 2021 |
URI: | https://ir.uitm.edu.my/id/eprint/61064 |
Download
![[thumbnail of 61064.pdf]](https://ir.uitm.edu.my/style/images/fileicons/text.png)
61064.pdf
Download (44kB)
Digital Copy
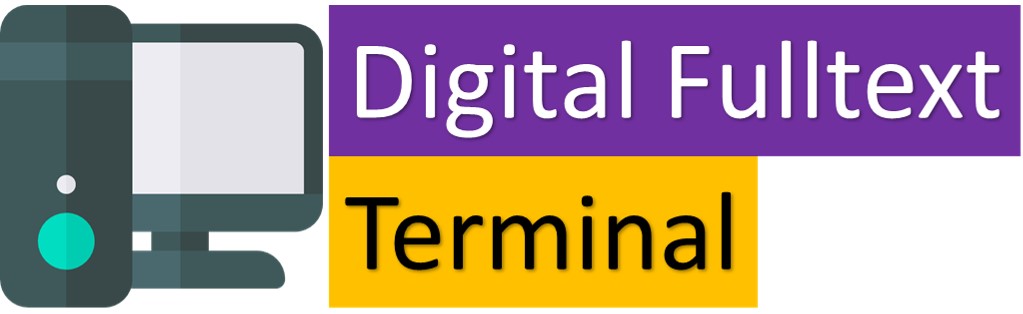
Physical Copy
ID Number
61064
Indexing
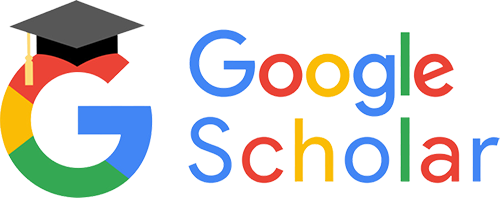
