Abstract
Clinical Decision Support System (CDSS) is a health information system, which is designed to help physicians in decision-making process. CDSS was introduced as one of the most effective ways in reducing medication errors and improving healthcare quality. Nevertheless, it remains a challenge to successfully provide and implement CDSS in practice. CDSS can be classified into two main categories based on the techniques used to develop the reasoning engine. The data driven CDSS are based on machine learning, while knowledge-based CDSS are based on logic-based techniques (e.g. inference rules and ontology). Each CDSS approach has its own strengths and limitations. The knowledge-based approach able to provide comprehensible knowledge, but it depends heavily on domain expert’s intervention and becomes a main bottleneck in forming its knowledge base. The data-driven approach reduces the need for expert input, as domain knowledge can be acquired and learned from clinical data, but its black-box learning process causes difficulties in understanding the system behaviour and knowledge represented. However, both of these CDSS approaches play important roles in developing an efficient CDSS. Therefore, the fusion of these approaches will extract the strengths from both of them, and consequently improving system efficiency. On the other hand, there are issues of limited provisions of causal reasoning and evidences in existing CDSS. These are necessary for showing the relevance and reliability of the system outcomes, and help the physicians in making proper decisions. As a result, this study proposed a CDSS model that consists of two modules: Neuro-Fuzzy Learning and Semantic Causal Reasoning modules. The Neuro-Fuzzy Learning Module represents the use of Adaptive Neuro-Fuzzy Inference System (ANFIS) for developing the model and making predictions. ANFIS is a hybrid of machine learning (i.e. neural network) and knowledge-based (i.e. fuzzy logic) techniques. ANFIS has the advantage of knowledge learning capabilities, which is hybrid learning from both patient data and expert knowledge. This advantage facilitates the CDSS to be adaptive to clinical practice and provide support to evidence-based medicine. The Semantic Causal Reasoning Module represents the use of ontology for modelling and integrating the concepts of medical domain knowledge with causal reasoning, decision support, and supporting evidences. These concepts are related with each other for providing a comprehensive description and explanation for represented knowledge. The CDSS model is developed for clinical cases of Methadone Maintenance Therapy (MMT). The developed ANFIS model is validated with real MMT patient’s data, where the training data is used to build the system, while testing data is for testing the system’s performance. For the ontology evaluation, several tools are used including the Ontology Pitfall Scanner, ontology metrics and competency questions. The results demonstrate that the CDSS model is feasible as the ANFIS performances show a good fit in both training and testing sets. The ontology model contains good quality and more expressive compared to other models. Most of information represented by the ontology model was accepted and acknowledged by the MMT physicians / experts. We also compared the features of our CDSS model with some of existing CDSS models. It shows that our CDSS model able to provide full support and fulfil the requirements for provision of causal explanation and evidence compared to other CDSS models. These signify the contributions of this study and the CDSS model has potential to be used as an aid for the physicians in making proper decisions and reducing medical errors in practice.
Metadata
Item Type: | Thesis (PhD) |
---|---|
Creators: | Creators Email / ID Num. Rahim, Nur Raidah 2012660438 |
Contributors: | Contribution Name Email / ID Num. Thesis advisor Nordin, Sharifalillah (Dr.) UNSPECIFIED |
Subjects: | Q Science > QA Mathematics > Instruments and machines > Electronic Computers. Computer Science > Expert systems (Computer science). Fuzzy expert systems R Medicine > R Medicine (General) > Computer applications to medicine. Medical informatics |
Divisions: | Universiti Teknologi MARA, Shah Alam > Faculty of Computer and Mathematical Sciences |
Programme: | Doctor of Philosophy (Information Technology and Quantitative Sciences) |
Keywords: | Health information system; Clinical Decision Support System (CDSS) |
Date: | August 2020 |
URI: | https://ir.uitm.edu.my/id/eprint/60922 |
Download
![[thumbnail of 60922.pdf]](https://ir.uitm.edu.my/style/images/fileicons/text.png)
60922.pdf
Download (41kB)
Digital Copy
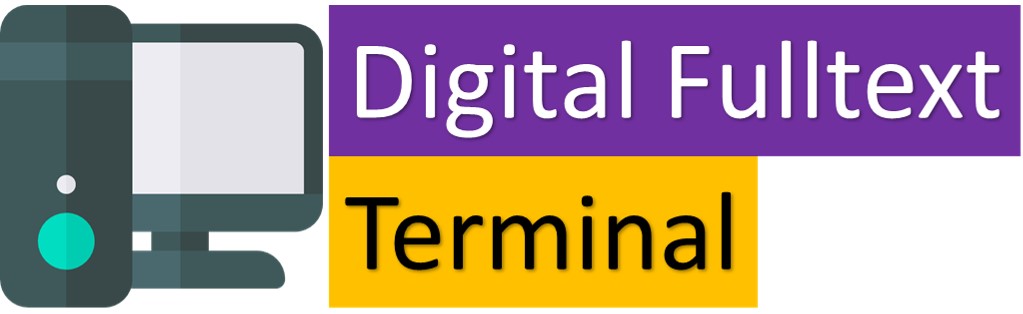
Physical Copy
ID Number
60922
Indexing
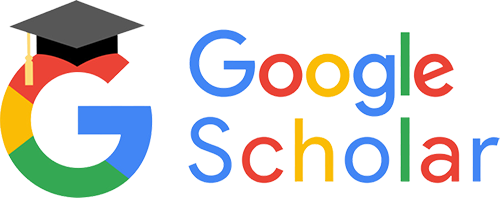
