Abstract
Composite material failure has been studied extensively for many years. However, the failure behaviour of composite materials that spontaneously fail is challenging to analyse. Hybrid composite is one of the recommended methods in modern construction to improve composites unexpected failure mode. However, because failure behaviour of hybrid composite laminates demands a lot of data, it must be predicted properly. This study's purpose is to predict the failure of hybrid composite laminates under uniaxial tension using artificial neural networks. Changing the angle of fibre orientation changed the failure behaviour of composite laminates. ANSYS Mechanical APDL 2020 was used to construct FE models to simulate physical testing. It was predicted using the ANSYS standard formulation and Maximum Stress Failure Criteria. On had already checked the model's results against acceptable public results. Using simply supported composite plates, uniaxial tension was studied. The plate has 24 layers and a layup of [0,0]. The FPF load represents the load required to attain the study's failure condition. Angle fibre orientation may affect the failure mode of composite laminates in uniaxial stress. The ANN tool in MATLAB predicted the failure. Finally, the ANSYS APDL data failure analysis was compared to the ANN model failure data. For Glass/Epoxy, Graphite/Epoxy, and Boron/Epoxy, the output failure rates are 18.97%, 6.25%, and 4.04 percent, respectively. The results of the study revealed that the method approaches produced more realistic and reliable results, with FEA results closely matching the analytical results. The current study is notable in that it advances knowledge about predicting failure behaviour in hybrid composite laminates using artificial neural networks.
Metadata
Item Type: | Thesis (Masters) |
---|---|
Creators: | Creators Email / ID Num. Nanihar, Muhammad Nadiarulah 2019526959 |
Contributors: | Contribution Name Email / ID Num. Thesis advisor Mahmud, Jamaluddin (Professor Ir. Dr.) UNSPECIFIED |
Subjects: | Q Science > QC Physics > Composite materials T Technology > TJ Mechanical engineering and machinery |
Divisions: | Universiti Teknologi MARA, Shah Alam > College of Engineering |
Programme: | Master of Science (Mechanical Engineering) |
Keywords: | Composite material; micromechanical; classical lamination theory; artificial neural network |
Date: | September 2021 |
URI: | https://ir.uitm.edu.my/id/eprint/60254 |
Download
![[thumbnail of 60254.pdf]](https://ir.uitm.edu.my/style/images/fileicons/text.png)
60254.pdf
Download (246kB)
Digital Copy
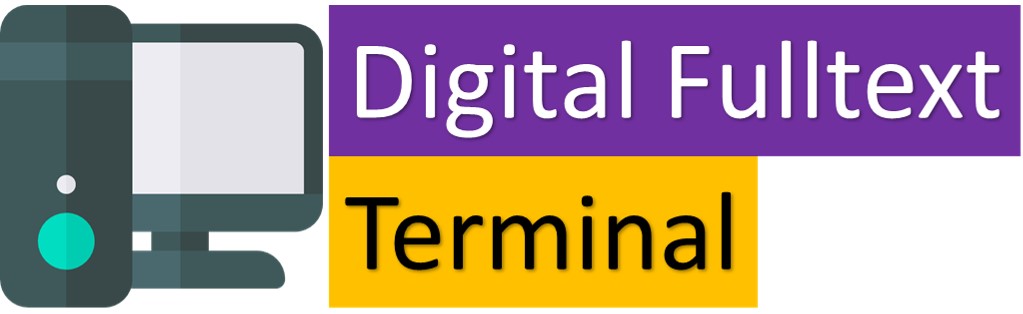
Physical Copy
ID Number
60254
Indexing
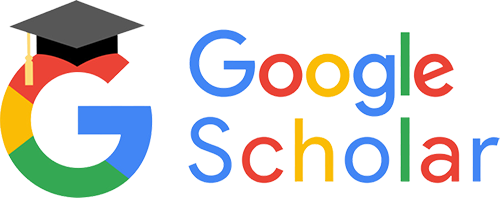
