Abstract
The screening of diabetic retinopathy (DR) affects the visual inspection of retina images
taken by ophthalmologists to detect the specific signs of pathology such as exudate,
haemorrhage (HEM) and microaneurysm (MA). Currently, this process is conducted
manually in many hospitals. Therefore, it is time-costing and risky for humans to make
mistake. An automatic and accurate system for detection DR signs can significantly
help ophthalmologists to make best decision for early treatment thus reducing risk of
vision loss. In general, this thesis introduces an automated machine learning algorithm
for detecting diabetic retinopathy (DR) in fundus images. To improve the performance
from current systems, this work has investigation on different of image pre-processing
enhancement technique to support accuracy on deep learning for DR classification. For
the image enhancement process, this thesis has proposed high-pass filter combined with
histogram equalization and de-haze algorithm respectively to improve the visual quality
of fundus images. Based on investigation different architecture and parameter, the
suitable deep learning model has been presented to get optimize best result and testing
time. To solving pattern classification problem, the optimization deep learning
architecture and parameter by using four convolution layers is set up to classify the three
pathological signs; HEM, MA and exudate. Two public online datasets of fundus image,
e-Ophtha and DIARETDB1 are used to evaluate the performance of this system. The
performance of the system demonstrates the effectiveness of image enhancement in
term of test accuracy percentage. From training and testing results using enhanced DR
images, the both pre-processing steps has proven slight improvement of deep learning
in the overall accuracy by 4.3% and 3.6% supported by enhancement 1 and 2
respectively, compared to those original images with no enhancement for both datasets.
Metadata
Item Type: | Thesis (Masters) |
---|---|
Creators: | Creators Email / ID Num. Abu Samah, Abdul Hafiz 2019995313 |
Contributors: | Contribution Name Email / ID Num. Compiler Ahmad, Fadzil (Dr.) UNSPECIFIED |
Subjects: | Q Science > QA Mathematics > Instruments and machines > Electronic Computers. Computer Science > Algorithms R Medicine > R Medicine (General) > Medical technology |
Divisions: | Universiti Teknologi MARA, Shah Alam > College of Engineering |
Programme: | Master of Science (Electrical Engineering) |
Keywords: | Diabetic retinopathy (DR); retina images; automated machine learning algorithm; deep learning model |
Date: | August 2021 |
URI: | https://ir.uitm.edu.my/id/eprint/60075 |
Download
![[thumbnail of 60075.pdf]](https://ir.uitm.edu.my/style/images/fileicons/text.png)
60075.pdf
Download (294kB)
Digital Copy
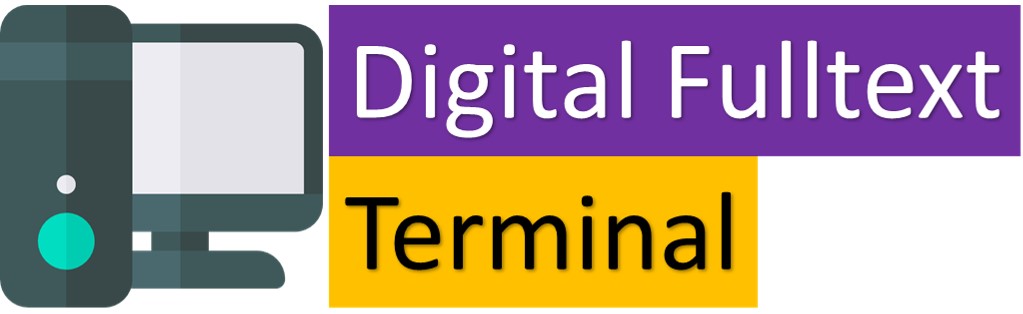
Physical Copy
ID Number
60075
Indexing
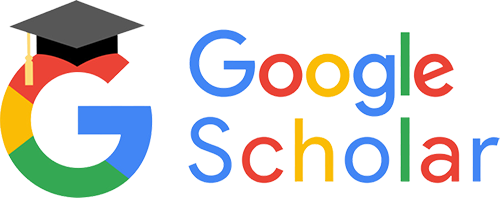
