Abstract
Globally, obese population prevalence is estimated using Body Mass Index (BMI) data from collected surveys and generally analyzed using simple linear regression method. Even the World Health Organization (WHO) uses this simple BMI index to define overweight and obesity. However, this BMI method of estimating national obesity population prevalence, although simple, is excessively costly involving huge amount of public health data. The aim of the study is to provide an alternatively cheaper obesity predictive analytics using dietary patterns to overcome the current exorbitantly high operation cost. Exploring the feasibility of using grocery data, these data are assessed from physical receipts collected from the selected household sampling which is generated into nutrition data, and then stored in a food engine (G2NE) developed in this study. Data mining technology is then applied on the tested nutrition data to run the obesity predictive analytics. In this study, BMI data are used as a complementary variable to nutrition variable in estimating the individual nutrition consumption, which are assigned as input data in testing. The prediction modelling is developed by conducting the processes in exploratory phase. The findings from the predictive analytics process in exploratory phase show that the use of 70:30 training and testing data split are the best test options for nutrition dataset based on Area Under the Curve (AUC) performance measurement. From the analysis, obese household shows the patterns that have higher intake percentage of processed food of food pyramid level three compared to non-obese household. The processes in the proposed prediction method involve three different domains which are retail, nutrition and health. In bridging the process involving these three domains, the obesity prediction method in this study is proposed in a form of a process framework (G2NOP Framework). This study has come out with two types of research contributions which are practical contributions from data pre-process phase as well as methodological contributions from the exploratory phase and framework integration phase. The limitations in this study include the nutrition assumptions and the scope of the respondents. Hence, the continuation of the future work is to extend the G2NE with the implementation of grocery and nutrition data sharing from the grocery retailers in which the use of larger scope of the household sampling should be considered.
Metadata
Item Type: | Thesis (PhD) |
---|---|
Creators: | Creators Email / ID Num. Daud, Nur'Aina 2014208596 |
Contributors: | Contribution Name Email / ID Num. Thesis advisor Mohd Noor, Nor Laila (Prof. Dr. ) UNSPECIFIED |
Subjects: | R Medicine > RC Internal Medicine > Specialties of internal medicine > Metabolic diseases > Obesity |
Divisions: | Universiti Teknologi MARA, Shah Alam > Faculty of Computer and Mathematical Sciences |
Programme: | Doctor of Philosophy (Information Technology) CS951 |
Keywords: | Obesity, Dietary, WHO |
Date: | April 2021 |
URI: | https://ir.uitm.edu.my/id/eprint/53621 |
Download
![[thumbnail of 53621.pdf]](https://ir.uitm.edu.my/style/images/fileicons/text.png)
53621.pdf
Download (180kB)
Digital Copy
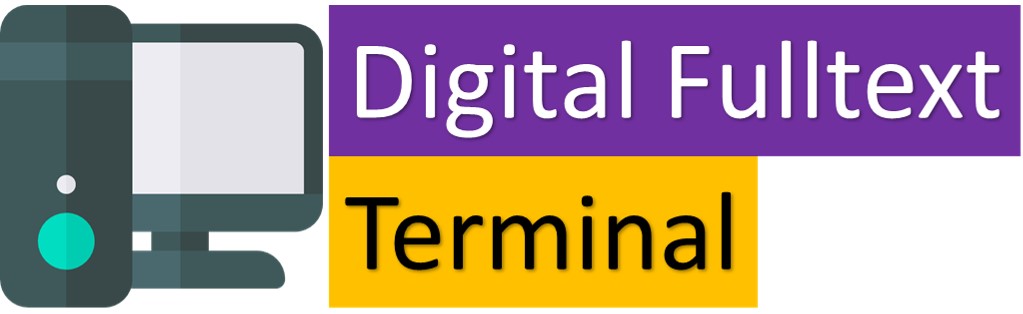
Physical Copy

ID Number
53621
Indexing
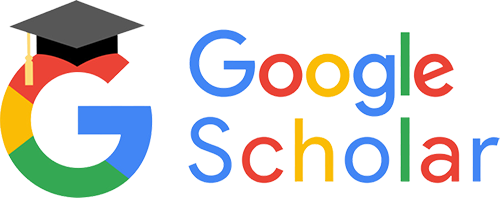
