Abstract
In Malaysia, various energy savings programmes have been introduced by the government and private entities to reduce energy consumption in buildings. For an organization to demonstrate the impact of an energy savings programme, the improvement in energy use performance should be properly evaluated and reported. Measurement and verification (M&V) procedure has been introduced worldwide for quantifying energy savings from energy efficiency programmes. In reporting accurate energy savings, a proper baseline energy model as specify in the M&V protocols must be developed. Linear regression (LR) technique being the common method to determine the baseline energy is less suitable for modelling the non-linear relationship of energy consumption and its variables, thus contributes errors to energy savings. Therefore, this study proposes several techniques to develop an accurate M&V baseline energy model for a retrofit chiller system in a commercial building using M&V Option B – retrofit isolation measurement method and energy savings programme in an educational building using Option C – whole facility. A dataset composed of three inputs of a chiller system in Option B; operating time, refrigerant tonnage, and differential temperature. On the other hand, working days, class days, and Cooling Degree Days (CDD) are used as input in Option C. The output from the models is electrical energy consumption. The baseline energy model was first developed using LR and Artificial Neural Network (ANN) for both measurement methods. Then, a properly trained ANN was used to establish the relation between the energy inputs and output for the case in Option B. Three metaheuristic techniques namely; Evolutionary Programming (EP), Particle Swarm Optimisation (PSO) and Artificial Bee Colony (ABC) are hybridised with ANN to improve the training process as well as to select the optimal values for ANN initial weights and biases. Secondly, the hybrid ANN baseline energy models were integrated with resampling techniques namely; Cross-Validation and Bootstrap technique to examine the ability of the ANN to deal with a small dataset particularly Option C that use monthly energy bill as the energy measurement data. Thirdly, a novel approach for the energy consumption planning is introduced by embedding the Artificial Bee Colony Hybrid Artificial Neural Network (ABCHANN) into an energy consumption optimisation model to minimise the predicted post-retrofit energy consumption. Results show that, the ANN baseline energy model has higher correlation than the LR technique hence providing a better performance in terms of mean squared error (MSE), mean absolute percentage error (MAPE), and standard error (SE). By hybridising the ANN with metaheuristic techniques has further improved the accuracy of ANN baseline model particularly the ABC technique by 2.31% which exhibited the best performance. Moreover, by integrating the resampling techniques with ANN for a small data set as in Option C has increased 10.08% accuracy of the baseline model. Comparing the two resampling techniques, the Cross-Validation technique combined with ABCHANN has the highest correlation and lower errors. In modelling the optimum post-retrofit energy consumption model, the ABCHANN outperformed the ANN in terms of predicting ability and accuracy. The ABCHANN post-retrofit energy consumption model is useful for energy consumption planning to minimize the energy use thus increase the potential savings by controlling the energy consumption’s variables in buildings.
Metadata
Item Type: | Thesis (PhD) |
---|---|
Creators: | Creators Email / ID Num. Wan Md Adnan, Wan Nazirah 2015223864 |
Contributors: | Contribution Name Email / ID Num. Thesis advisor Dahlan, Nofri Yenita (Assoc. Prof. Ir. Dr.) UNSPECIFIED |
Subjects: | T Technology > TJ Mechanical engineering and machinery > Power resources T Technology > TJ Mechanical engineering and machinery > Energy conservation |
Divisions: | Universiti Teknologi MARA, Shah Alam > Faculty of Electrical Engineering |
Programme: | Doctor of Philosophy (Electrical Engineering) – EE950 |
Keywords: | Energy, saving, artificial |
Date: | February 2021 |
URI: | https://ir.uitm.edu.my/id/eprint/46740 |
Download
![[thumbnail of 46740.pdf]](https://ir.uitm.edu.my/style/images/fileicons/text.png)
46740.pdf
Download (327kB)
Digital Copy
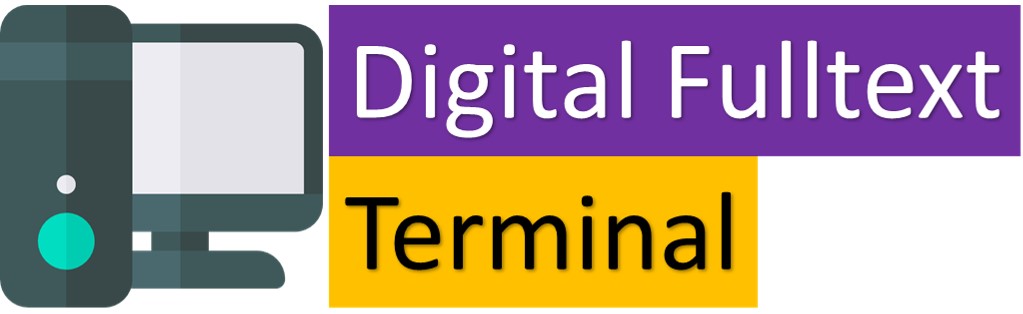

Physical Copy
ID Number
46740
Indexing
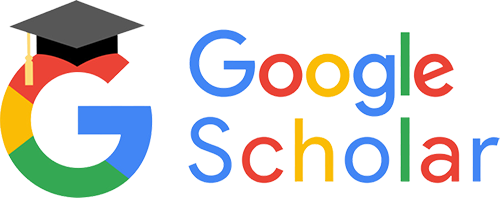
