Abstract
In this project, Hybrid Multilayer Perceptron (HMLP) neural network is used for system modeling to improve the speed and the convergence in training process. This project requires collecting of a raw material data from controlling devices Proportional Integral and Derivative (PID) control system from Modular Servo control system (MS150).The HMLP neural network program is designed using MATLAB. The HMLP network is trained using a Modified Recursive Prediction Error (MRPE) algorithm to obtain the appropriate parameter for the network. Based on the analysis of performance, the developed system is able to achieve high accuracy and minimum error. The accuracy is at the rate of 99.58%, while the error and Mean Square Error (MSE) are at the rate of 0.42% and 4.9840e-8. The analysis of the performance of the HMLP network has proven that it is suitable to be used in system modeling. The network strategy employed will result in fast speed of convergence rate if compared to the Multilayer Perceptron (MLP) network, but low speed in program running time because of the HMLP structure is more complex than MLP network. The HMLP network also indicates that the network models adequately represents the systems dynamic.
Metadata
Download
![[thumbnail of 43222.PDF]](https://ir.uitm.edu.my/style/images/fileicons/text.png)
43222.PDF
Download (93kB)
Digital Copy
Physical Copy

ID Number
43222
Indexing
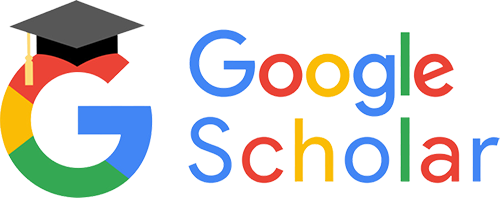
