Abstract
Parkinson’s disease (PD) is the second commonest late life neurodegenerative disease after Alzheimer’s disease. It is prevalent throughout the world and predominantly affects patients above 60 years old. It is caused by progressive degeneration of dopamine containing cells (neurons) within the deep structures of the brain called the basal ganglia and substantia nigra. Therefore, accurate prediction of PD need to be done in order to assist medical or bio-informatics practitioners for initial diagnose of PD based on variety of test results. This paper described the analysis conducted based on two training algorithms namely Levenberg-Marquardt (LM) and Scaled Conjugate Gradient (SCG) of Multilayer Perceptrons (MLPs) Neural Network in diagnosing PD with Analysis of Variance (ANOVA) as a feature selection. The dataset information of this project has been taken from the Parkinson Disease Data Set. Results attained confirmed that the LM performed well with accuracy rate of above 90% before and after feature selection whilst SSG attained above 85% subsequent to implementation of ANOVA as feature selection.
Metadata
Item Type: | Thesis (Masters) |
---|---|
Creators: | Creators Email / ID Num. Abu Bakar, Zahari UNSPECIFIED Ibrahim, Nur Farahiah UNSPECIFIED Ispawi, Dzufi Iszura UNSPECIFIED Md. Tahir, Nooritawati UNSPECIFIED |
Subjects: | R Medicine > R Medicine (General) > Medicine and disease in relation to psychology. Terminal care. Dying R Medicine > RA Public aspects of medicine > Medical care R Medicine > RC Internal Medicine > Neuroscience. Biological psychiatry. Neuropsychiatry > Neurology. Diseases of the nervous system. Including speech disorders R Medicine > RC Internal Medicine > Diseases of the brain |
Divisions: | Universiti Teknologi MARA, Sarawak > Kota Samarahan Campus > Faculty of Electrical Engineering |
Programme: | Master in engineering |
Keywords: | Parkinson’s, disease, Neural network |
Date: | December 2012 |
URI: | https://ir.uitm.edu.my/id/eprint/42965 |
Download
![[thumbnail of 42965.pdf]](https://ir.uitm.edu.my/style/images/fileicons/text.png)
42965.pdf
Download (263kB)
Digital Copy
Physical Copy

ID Number
42965
Indexing
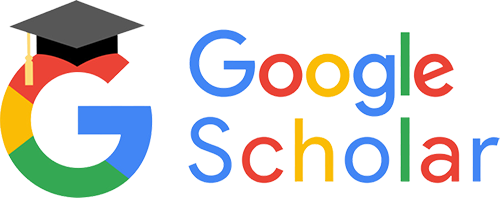
