Abstract
It is well known that the use of a gas sensor array and pattern recognition system offers an effective technique for the identification of volatile organic molecules because of the poor selectivity of a lot of other gas sensors. Among the chemometric techniques for signal processing, artificial neural networks (ANNs) are powerful tools, which have been employed in a diverse range of applications in recent years. This project focuses only on the development of an Artificial Neural Network (ANN) for the classification of Volatile Organic Compounds (VOCs). The ANNs that were used in this project were Multilayer Perceptron (MLP), Learning Vector Quantization (LVQ) and Adaptive Network Based Fuzzy Inference System (ANFIS). The types of VOCs used for the classification were Acetone, Benzene, Chloroform, Ethanol and Methanol. In this project, the networks were trained using certain types training algorithm depending on the types of networks; Levenberg Marquardt (LM) for the MLP, competitive network for the LVQ and hybrid learning for ANFIS. The input and output neurons used for the three networks were 5 neurons. The optimum structure of the neural network was determined by trial and error method to obtain the optimized hidden neuron and weight values. The performance of each neural network was analysed in terms of the classification accuracy, average score, total training time required during the training process and the test time of the optimised networks. From the results obtained, the classification accuracy and the average score percentages for the MLP network was the highest (above 90%). The ANFIS network was also observed to have good classification rate with classification accuracy and average score percentage above 90%. The LVQ network was observed to have poor classification for the VOCs data with classification accuracy percentage below 80%. The training time for ANFIS network was the shortest (361.14 seconds) which was then followed by the MLP (536.27 seconds) and LVQ networks (5427.35 seconds). However, the test time for MLP network was the shortest (0.0108 second) which was then followed by the LVQ network (0.0133 second) and ANFIS network (0.078 second). Overall, the MLP was considered the best optimised network followed by ANFIS and LVQ networks.
Metadata
Item Type: | Research Reports |
---|---|
Creators: | Creators Email / ID Num. Noorsal, Emilia UNSPECIFIED Osman, Muhammad Khusairi UNSPECIFIED Mokhtar, Norfadzilah UNSPECIFIED |
Subjects: | T Technology > TD Environmental technology. Sanitary engineering > Special types of environment. Including soil pollution, air pollution, noise pollution T Technology > TD Environmental technology. Sanitary engineering > Special types of environment. Including soil pollution, air pollution, noise pollution > Malaysia |
Divisions: | Universiti Teknologi MARA, Pulau Pinang > Permatang Pauh Campus |
Keywords: | Artificial Neural Networks (ANNs), Volatile Organic Compounds (VOCs), ANFIS |
Date: | June 2007 |
URI: | https://ir.uitm.edu.my/id/eprint/39952 |
Download
![[thumbnail of 39952.PDF]](https://ir.uitm.edu.my/style/images/fileicons/text.png)
39952.PDF
Download (128kB)
Digital Copy
Physical Copy

ID Number
39952
Indexing
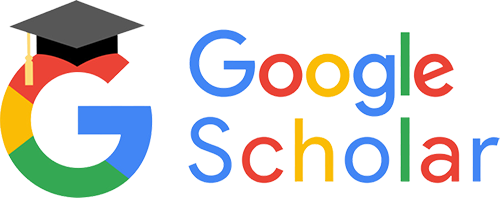
