Abstract
This project discuss the performance of evaluating windowing based continuous S-Transform with deep learning classifier for detection and classifying interrupt and transient disturbances. The primary purpose was to analyse the detection and classification of voltage interrupt and transient using S-Transform (ST) as signal processing technique. The detection technique was divided into half-cycle and one- cycle windowing technique (WT), both cycle is used for the purpose of comparison. The disturbances signal was create using MATLAB programming language and set in form of m-file. S-Transform (ST) was used to extract the significant feature in a form of scattering data from disturbances signal. Then, the scattering data was used to build the detection interface inside the disturbances signal. The scattering data also was actual an input for extreme learning machine neural network (ELMNN) in order to classify the percentage accuracy of the disturbance signal. In addition, this analysis was verified that by using windowing technique of half-cycle and one-cycle it can provide clear detection in detecting the existent of disturbance within electrical distribution signal. Extreme learning machine was one of the suitable neural network to be used in classifying power quality disturbance due to its advantage in processing large dataset and able to produces high percentages accuracy. This project also includes 3 type of different sample for each disturbance which were interrupt and transient for the comparison purpose.
Metadata
Download
![[thumbnail of 39879.pdf]](https://ir.uitm.edu.my/style/images/fileicons/text.png)
39879.pdf
Download (244kB)
Digital Copy
Physical Copy
ID Number
39879
Indexing
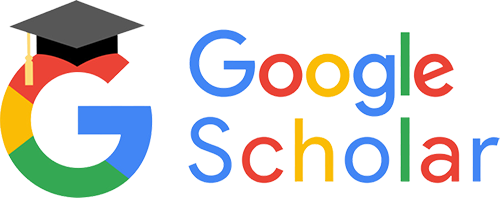
