Abstract
Autism Spectrum Disorder (ASD) is a complex neurobehavioral condition that disrupts the growth of one’s mentality by affecting their actions and communications. In dealing with ASD students in a classroom, there are several problems faced by educators such as catering the appropriate method for the student’s educational needs in a classroom setting. This is due to the student’s behaviour in the disorder that tends to mask their capabilities and intelligence. Previous studies also indicates that school administrations and educators find it a challenging task in class placements for autistic students due to the lack of information regarding the autistic student’s needs. Thus, this project proposes a solution to the problems by utilizing the machine learning approach which is the Agglomerative clustering algorithm. Previous studies shows that homogenous grouping of autistics students yields positive results, therefore, this project proposes to design and develop a clustering model system known as the CASDSS (Clustering Autism Spectrum Disorder Students System) where the main goal of this system is to create a homogenous grouping of the ASD students based on their behaviour, skills and intelligence. Another feature that the CASDSS offers is visualization where the system is able to visualize the clustering results as well as the strengths and weaknesses of the ASD students. 80 data regarding the autistic student’s behaviour, intelligence and skills are collected from special schools through distribution of questionnaires. The data that are collected comprises of 27 attributes where 13 of the attributes are regarding the student’s behaviour, 6 attributes regarding their skills and 6 regarding the student’s academics. Data cleaning and data transformation is first carried out, followed by normalization through the Z-score method before being processed in the clustering model. Several charts such as dendrograms and sunburst charts are used to visualize the clustering results. The CASDSS is then tested through functionality and usability testing to ensure that system runs without any error and to obtain the targeted user’s feedback in utilizing the system. To conclude, it is found that the Agglomerative Clustering approach in the model is able to solve the challenge in identifying the student’s educational need and the system is able to visualize the student’s strength and weaknesses in their academics, skills and behaviour. Hence, based on the model in the system developed, all objectives are achieved. As an extension in the study, future recommendations should include a classifying model that can classify ASD student’s method and approach in teaching them.
Metadata
Item Type: | Thesis (Degree) |
---|---|
Creators: | Creators Email / ID Num. Nordin, Daarin Nadia 2017412216 |
Contributors: | Contribution Name Email / ID Num. Thesis advisor Abd Halim, Khairul Nizam UNSPECIFIED |
Subjects: | Q Science > QA Mathematics > Instruments and machines Q Science > QA Mathematics > Instruments and machines > Electronic Computers. Computer Science Q Science > QA Mathematics > Instruments and machines > Electronic Computers. Computer Science > Algorithms |
Divisions: | Universiti Teknologi MARA, Melaka > Jasin Campus > Faculty of Computer and Mathematical Sciences |
Programme: | Bachelor of Computer Science (Hons) (CS230) |
Keywords: | Autism Spectrum Disorder (ASD); Student’s behaviour; Agglomerative clustering algortihm |
Date: | 2020 |
URI: | https://ir.uitm.edu.my/id/eprint/35501 |
Download
![[thumbnail of 35501.pdf]](https://ir.uitm.edu.my/style/images/fileicons/text.png)
35501.pdf
Download (154kB)
Digital Copy
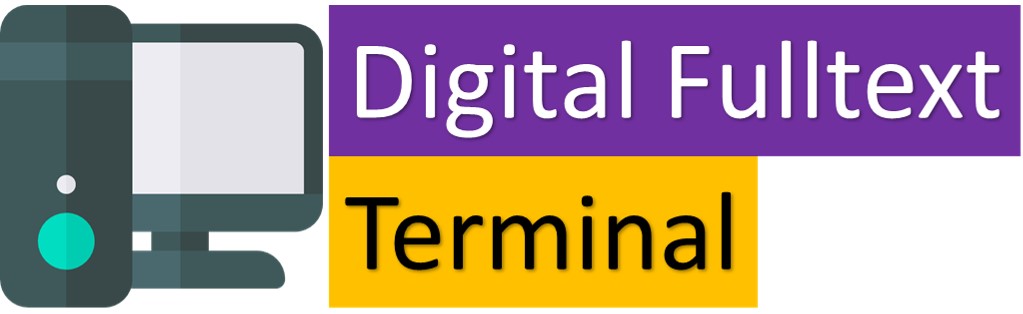
Physical Copy
ID Number
35501
Indexing
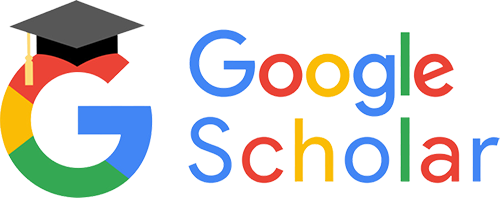
