Abstract
This thesis presents a novel analysis and classification of human body electromagnetic radiation (EMR) for post stroke patient and non-stroke participant. Presently, some researchers are investigating the human body EMR to characterize the physiological aspect of human being. Some methods include Kirlian photography, gas discharge visualization (GDV) and polycontrast interference photography. However, there are some drawbacks in the current techniques such as the images are not reliable and distorted. Moreover, the relationship between human body EMR and the health condition of post stroke patients has not been found. Thus, this research aims to scientifically establish a fundamental idea and new non-invasive technique to identify and to differentiate human body EMR of post stroke patients and non-stroke participants. The participants' body frequencies are captured at 16 points around the human body and 7 points of Chakra System using a frequency detector. The first part of the analysis concerned with the EMR of the whole body while the second part extended and zooming into specific body segments of chakra, left side, right side, upper body, middle body and lower body. Initially, the characteristics of frequency radiation are examined using statistical analysis to find the correlations between variables, to examine differences of frequency radiation characteristics between samples and to explore the relationship among variables. Next, the classification algorithm of k-nearest neighbor (KNN) and artificial neural network (ANN) are applied to discriminate between the samples and between their body segments. The classifiers are evaluated through analysis of the performance indicators of confusion matrix consisting of accuracy, precision, specificity and sensitivity. The findings of this research show that the EMR characteristics of the samples are different. A successful classification is produced in KNN and ANN classification for post stroke and non-stroke recognition, which is in line with the statistical analysis calculated. In general, the performance measure of training consisting of accuracy, sensitivity, specificity and precision for KNN classification achieved 100% while for ANN achieved 98% - 100%. In addition, for KNN performance measure of testing achieved 77% - 100% while for ANN achieved 75% - 92%. For body segment recognition, the classification results of both classifiers range between 69% - 100% for training accuracy and 64%-92% for testing accuracy. The outcomes of the classifier show that it is able to classify the human body EMR using KNN and ANN analysis. As a conclusion, the KNN classifier exhibit better results compared to ANN. This finding confirmed that the EMR of human body has different characteristics between samples of post stroke and non-stroke and between their body segments.
Metadata
Item Type: | Thesis (PhD) |
---|---|
Creators: | Creators Email / ID Num. S. Abdul Kadir, Ros Shilawani UNSPECIFIED |
Subjects: | Q Science > QC Physics > Radiation physics (General) |
Divisions: | Universiti Teknologi MARA, Shah Alam > Faculty of Electrical Engineering |
Programme: | Doctor of Philosophy in Electrical Engineering |
Keywords: | Electromagnetic, Physiological characteristic, Stroke |
Date: | 2018 |
URI: | https://ir.uitm.edu.my/id/eprint/27941 |
Download
![[thumbnail of 27941.pdf]](https://ir.uitm.edu.my/style/images/fileicons/text.png)
27941.pdf
Download (3MB)
Digital Copy
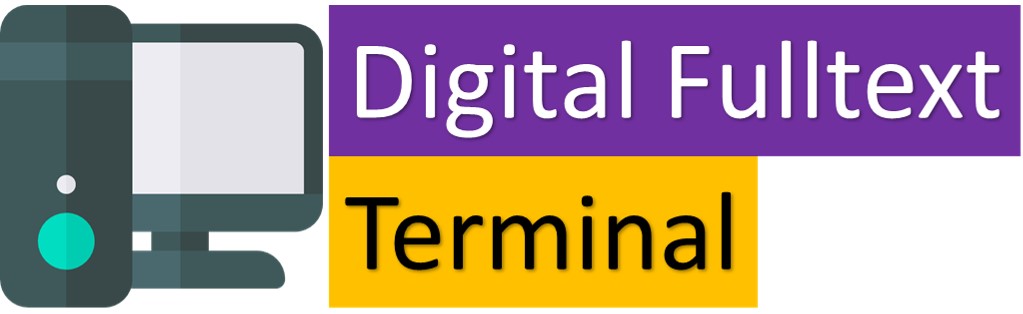

Physical Copy
ID Number
27941
Indexing
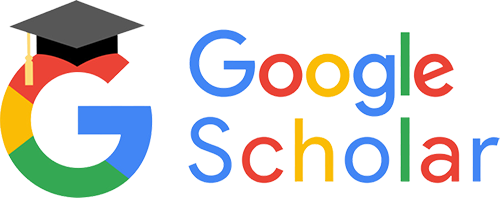
