Abstract
Infant asphyxia is a condition caused by inadequate intake of oxygen suffered by newborn babies. Early diagnosis of asphyxia is important to avoid complications such as damage to the brain, organ and tissue or even death. Asphyxia occurs in infants with neurological level disturbance, which is found to affect sound of cry produced by infants. The infant cry signals with asphyxia have distinct patterns which can be recognized with pattern classifiers such as Artificial Neural Network (ANN). This study investigates the performance of feature selection techniques namely F-Ratio, Orthogonal Lest Square (OLS) and Binary Particle Swarm Optimisation (BPSO) analysis in selecting optimal features extracted from feature extraction technique; Mel Frequency Cepstrum Coefficient (MFCC). Mel Frequency Cepstrum Coefficient (MFCC) was employed to extract the significant features from infant cry. The selected MFCC features were then used to train several ANN Multi-Layer Perceptron (MLP). The simulation results showed each method is able to improve classifier performance. Among three method discusses, BPSO was the best feature selection method with 96.03% classification accuracy followed by OLS (94%) and F-Ratio (93.38%).
Metadata
Item Type: | Thesis (Masters) |
---|---|
Creators: | Creators Email / ID Num. Zabidi, Azlee 2009809422 |
Contributors: | Contribution Name Email / ID Num. Thesis advisor Mansor, Wahidah UNSPECIFIED |
Subjects: | T Technology > TK Electrical engineering. Electronics. Nuclear engineering > Applications of electric power |
Divisions: | Universiti Teknologi MARA, Shah Alam > Faculty of Electrical Engineering |
Programme: | Master of Science |
Keywords: | Mel Frequency Cepstrum Coefficient, Binary Particle Swarm Optimization, multi-layer perceptron, asphyxia diseases |
Date: | 2012 |
URI: | https://ir.uitm.edu.my/id/eprint/20425 |
Download
![[thumbnail of 20425.pdf]](https://ir.uitm.edu.my/style/images/fileicons/text.png)
20425.pdf
Download (121kB)
Digital Copy
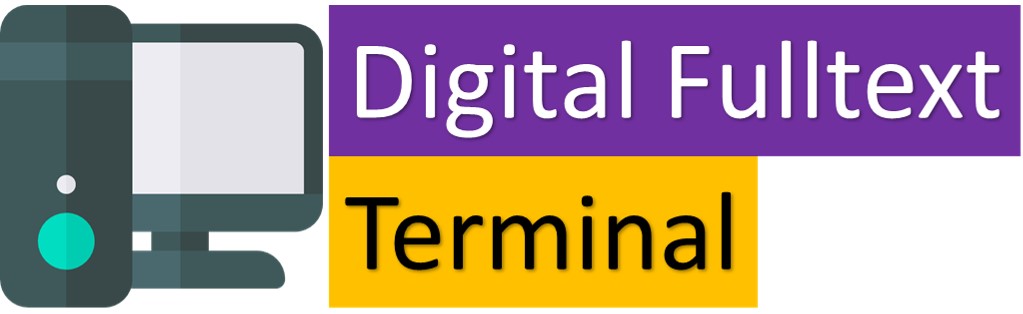
Physical Copy

ID Number
20425
Indexing
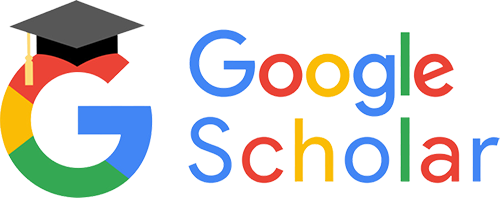

Statistic
