Abstract
System Identification (SI) is a control engineering discipline concerned with the discovery of mathematical models based on dynamic measurements collected from the system. It is an important discipline in the construction and design of controllers, as SI can be used for understanding the properties of the system as well as to forecast its behavior under certain past inputs and/or outputs. The NARMAX model and its derivatives (Nonlinear Auto-Regressive with Exogenous Inputs (NARX) and Nonlinear Auto-Regressive Moving Average (NARMA)) are powerful, efficient and unified representations of a variety of nonlinear models. The identification process of NARX/NARMA/NARMAX involves structure selection and parameter estimation, which can be simultaneously performed using the widely accepted Orthogonal Least Squares (OLS) algorithm.
Metadata
Item Type: | Book Section |
---|---|
Creators: | Creators Email / ID Num. Mohd Yassin, Ahmad Ihsan UNSPECIFIED |
Subjects: | L Education > LB Theory and practice of education > Higher Education > Dissertations, Academic. Preparation of theses > Malaysia |
Divisions: | Universiti Teknologi MARA, Shah Alam > Institut Pengajian Siswazah (IPSis) : Institute of Graduate Studies (IGS) |
Series Name: | IPSis Biannual Publication |
Volume: | 6 |
Number: | 6 |
Keywords: | Abstract; Abstract of thesis; Newsletter; Research information; Doctoral graduates; IPSis; IGS; UiTM; algorithm |
Date: | 2014 |
URI: | https://ir.uitm.edu.my/id/eprint/19441 |
Download
ABS_AHMAD IHSAN MOHD YASSIN TDRA VOL 6 IGS_14.pdf
Download (395kB) | Preview
ID Number
19441
Indexing
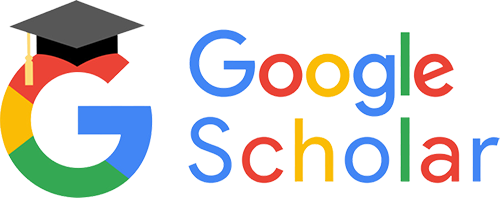
