Abstract
This thesis describes the development of Electrical Engineering students' performance prediction model using Artificial Neural Network (ANN) based on SIMS data from three generations of Matriculation and Diploma students. It was observed that there was a certain pattern or trend between the strong ability students and the weaker ones in terms of performance. The strong ability students managed to graduate steadily with high CGPA upon graduation, while the weaker ones tend to waver and finally graduate with minimum CGPA or even extended for another one or two semesters to complete the required credit hours. The Grade Points (GP) of fundamental subjects attempted at semester one was used as inputs to the developed Neural Network Students' Performance Prediction Model (NNSPPM) to predict the output which is CGPA8 upon graduation. The fundamental subjects strongly influenced the overall performance of students. The NNSPPM was then tested with another set of input data consisting GP of subjects at semester three to see the predicted output. The NNSPPM was further validated with a different set of data, namely Diploma students taking the same subjects at semester three, sitting the same set of examination questions as that of Matriculation students. The trend and pattern of predicted output seemed to hold true for all three different cases. It was found that at lower CGPA8, the predicted output is higher than the actual CGPA8; while at high CGPA8, the predicted is lower than the actual CGPA8 for the Matriculation and Diploma students. Subsequently a second method, Linear Regression was used to predict the final CGPA. GP of subjects scored by students form the independent while the CGPA8 formed the dependent variable. However, when the coefficient of Correlation R was compared between the two methods, NN method was found to be more accurate in terms of prediction. The Mean Square Error or Residual is almost the same in both methods. Thus the fundamental subjects at semester one or three have direct impact on CGPA8. The fundamental subjects strongly influenced performance of students. By using the prediction model, strategic intervention by the academic advisors can be offered to the underachieving students once detected by the model.
Metadata
Item Type: | Thesis (PhD) |
---|---|
Creators: | Creators Email / ID Num. Mohd Arsad, Pauziah 2011350117 |
Contributors: | Contribution Name Email / ID Num. Thesis advisor Buniyamin, Norlida nbuniyamin@uitm.edu.my |
Subjects: | L Education > LB Theory and practice of education > Performance. Competence. Academic achievement Q Science > QA Mathematics > Instruments and machines > Electronic Computers. Computer Science > Neural networks (Computer science) |
Divisions: | Universiti Teknologi MARA, Shah Alam > Faculty of Electrical Engineering |
Programme: | Doctor of Philosophy in Electrical Engineering (EE990) |
Keywords: | Neural network; Linear regression |
Date: | January 2016 |
URI: | https://ir.uitm.edu.my/id/eprint/18575 |
Download
![[thumbnail of 18575.pdf]](https://ir.uitm.edu.my/style/images/fileicons/text.png)
18575.pdf
Download (563kB)
Digital Copy
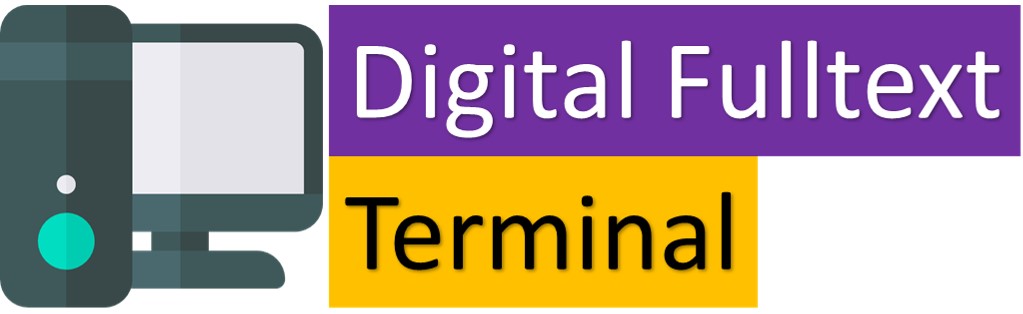

Physical Copy
ID Number
18575
Indexing
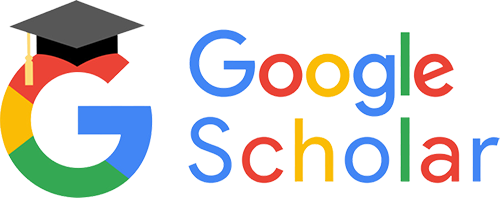
