Abstract
Economic Dispatch is an important component in power system planning and operation. This will lead to the capability of such power transmission in the utility system to perform their planning of power delivery to the consumer with minimal cost and acceptable emission level. Thus, a reliable and robust optimisation algorithm will be a priori in ensuring a profitable operation of the utility. Non-optimal values of generated power in a power system network may cause non-economic phenomena in terms of cost of generation and emission level. Thus a reliable optimisation approach is crucial to ensure the economic operation of the power system. The practice of only considering a single objective may affect the operation since only one objective function is considered. Thus, multi-objective needs to be considered to address this issue which considers more than one objective function. No effort on the possible prediction process may cause limited information on ED ranges. Thus, special efforts need to be conducted to forecast several possible scenarios under the variations of the input variables in ED studies. This thesis presents the Hybrid Evolutionary-Barnacles Mating Optimisation-Artificial Neural Network Based Technique for Solving Economic Power Dispatch Planning and Operation. In this study, a new optimisation algorithm termed Hybrid Evolutionary-Barnacles Mating Optimisation (HEBMO) was initially formulated to solve optimisation problems. The proposed HEBMO optimisation algorithm was employed to solve the convex and non-convex economic dispatch problems. Subsequently, a new multi-objective optimisation technique named Multi-Objective Hybrid Evolutionary-Barnacles Mating Optimisation (MOHEBMO) was developed to solve the minimization problems involving the total generation cost and total emission of harmful gasses in a multi-objective mode. Subsequently, the proposed HEBMO was integrated with an artificial neural network (ANN) for the scheduling of input features of the generator to predict total fuel cost and emission in power system planning. This will allow the power system planners and operators to use the relevant results for consumption at their control center. The first two proposed algorithms are validated on 2 reliability test systems (RTS) to represent a small and medium power transmission model namely the IEEE 30-Bus RTS and IEEE 57-Bus RTS. The third algorithm was validated on IEEE 30-Bus RTS only. Comparative studies were conducted concerning the traditional Evolutionary Programming (EP) and Barnacles Mating Optimiser (BMO) for performance evaluation of HEBMO and MOHEBMO. It is able to minimise the total generation cost and total emission. Integration of HEBMO and ANN has also indicated a great prediction of total generation cost and total emission while satisfying the optimal demand for power generation. This new model was compared with the ANN model for performance evaluation. A comparative study with respect to the traditional ANN revealed that the integration between HEBMO and ANN is much more accurate, indicated by the high value of the correlation coefficient, close to 1.00. All the proposed HEBMO, MOHEBMO, and HEBMO-ANN can be further applied in a larger system within the same research discipline. It is also feasible for application in solving other problems involving optimisation process and prediction.
Metadata
Item Type: | Thesis (PhD) |
---|---|
Creators: | Creators Email / ID Num. Ismail, Nor Laili 2019868418 |
Contributors: | Contribution Name Email / ID Num. Thesis advisor Musirin, Ismail UNSPECIFIED |
Subjects: | T Technology > TK Electrical engineering. Electronics. Nuclear engineering > Electric power distribution. Electric power transmission |
Divisions: | Universiti Teknologi MARA, Shah Alam > College of Engineering |
Programme: | Doctor of Philosophy (Electrical Engineering) |
Keywords: | Electrical energy, distribute electrical energy, hybrid evolutionary-barnacles mating optimisation (HEBMO) |
Date: | 2024 |
URI: | https://ir.uitm.edu.my/id/eprint/107142 |
Download
![[thumbnail of 107142.pdf]](https://ir.uitm.edu.my/style/images/fileicons/text.png)
107142.pdf
Download (378kB)
Digital Copy
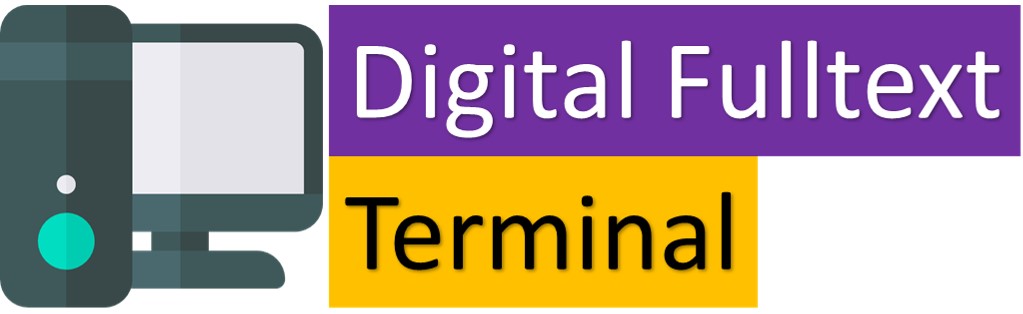
Physical Copy
ID Number
107142
Indexing
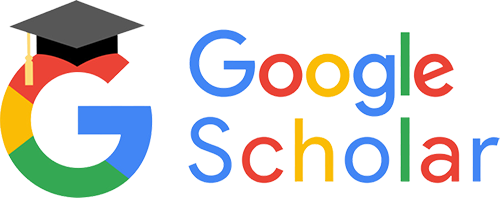
