Abstract
The topic of harmonics has received increased attention over the pass several years due to the increased installation of harmonic-producing that is harmonic-sensitive equipment. It is one of the most common power quality problems. Power quality is an increasing concern for utilities and their commercial and industrial electrical power users. This thesis provides multi-layered network based methods that is back propagation technique for predicting voltage harmonics in eight-bus industrial power system when two types of filter, single tune filter and high pass filter, are added to the certain bus-bar. In this thesis, the data for voltage harmonics in each bus has been verified by means of the computer simulation using Software for Power System (SPS) by Micromatrix Research Corporation. The result obtained from this experiment showed that this method of predicting the voltage harmonics has the advantage, that it can determine the voltage harmonics with very low error.
Metadata
Item Type: | Thesis (Degree) |
---|---|
Creators: | Creators Email / ID Num. Md. Aspan, Rosnita UNSPECIFIED |
Contributors: | Contribution Name Email / ID Num. Thesis advisor Hamzah, Noraliza UNSPECIFIED |
Subjects: | T Technology > TK Electrical engineering. Electronics. Nuclear engineering > Electronics > Apparatus and materials |
Divisions: | Universiti Teknologi MARA, Shah Alam > Faculty of Electrical Engineering |
Programme: | Bachelor of Electrical Engineering (Honours) |
Keywords: | Artificial neural network, Voltage harmonic |
Date: | 1999 |
URI: | https://ir.uitm.edu.my/id/eprint/104648 |
Download
![[thumbnail of 104648.pdf]](https://ir.uitm.edu.my/style/images/fileicons/text.png)
104648.pdf
Download (104kB)
Digital Copy
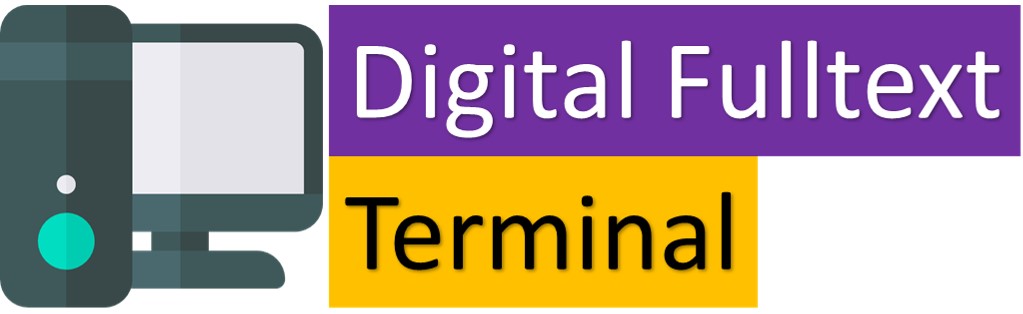
Physical Copy
ID Number
104648
Indexing
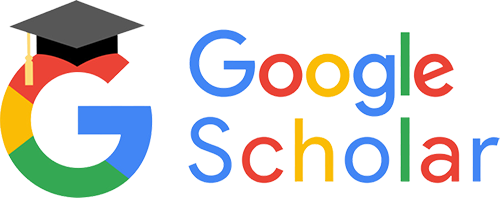
