Abstract
Load forecasting plays an important role in electric power system operations and planning purposes. Improving the accuracy of the load forecast can optimize the operation of the electrical system and save significant amount of money. Therefore, this study investigates the effectiveness of an artificial neural network (ANN) approach to short term load forecasting (STLF) in power systems. Two approaches of ANN algorithms i.e. the Back-Propagation Network (BPN) and Modular Neural Network (MNN) were investigated. Accuracy of the networks depend on some associated factors which usually affect ANN structure and training parameters such as learning rate (TJ), momentum constant (a), number of hidden node (s) and number of iteration. Hourly loads (24 hour per day) of current day were used as the inputs with the type of day of weekdays (Monday to Friday), Saturday and Sunday. The 24-hour loads for the next day were chosen as the outputs. Both networks were trained and tested on actual load data and weather conditions for the next day's load forecast by using software package Professional II/PLUS and NeuralWorks. The capability of both networks based STLF has been found to be very encouraging
Metadata
Item Type: | Thesis (Degree) |
---|---|
Creators: | Creators Email / ID Num. Rahim, Norhayati 96628144 |
Contributors: | Contribution Name Email / ID Num. Thesis advisor Hamzah, Noraliza UNSPECIFIED |
Divisions: | Universiti Teknologi MARA, Shah Alam > Faculty of Electrical Engineering |
Programme: | Bachelor of Engineering (Honors) |
Date: | 1999 |
URI: | https://ir.uitm.edu.my/id/eprint/103342 |
Download
![[thumbnail of 103342.pdf]](https://ir.uitm.edu.my/style/images/fileicons/text.png)
103342.pdf
Download (130kB)
Digital Copy
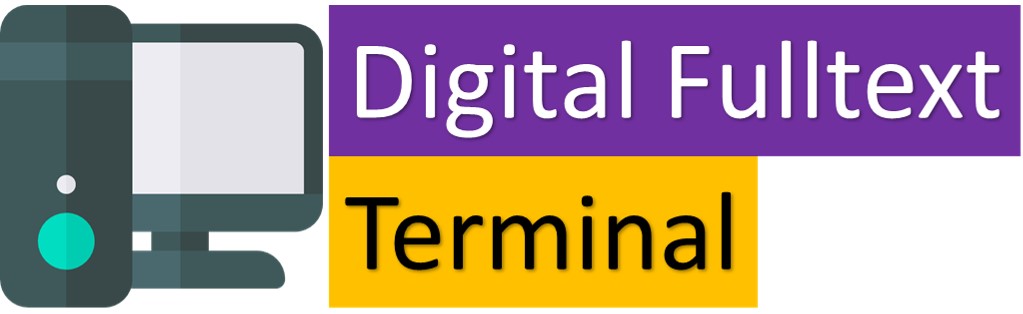
Physical Copy
ID Number
103342
Indexing
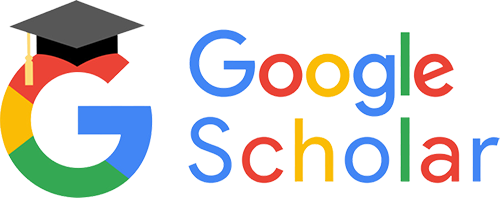
