Abstract
The aim of this thesis is to develop a Parkinson gait recognition technique that is able to evaluate and distinguish gait deviations experienced by Parkinson Disease (PD) patients from normal pattern. The research can be divided into two phase namely gait analysis of PD as compared to normal subjects, followed by gait classification using machine learning approach. Firstly, two types of statistical test are conducted which are independent t-test and Pearson’s correlation test. Raw gait database which consist of four basic gait features, five kinetic gait features and also twelve kinematic gait features are acquired from prior walking experiments of both PD and normal subjects. Based on statistical analysis conducted, significant different between PD and normal gait pattern are observed for four features, which are the step length and walking speed from basic features, maximum extension of hip from kinematic feature and maximum horizontal push-off force from kinetic feature. Hence these significant features are appropriate to be utilized for recognition of PD gait. Next, Principal Component Analysis (PCA) is used as feature extraction for each gait features from basic, kinetic and kinematic parameter followed by normalization based on intragroup as well as inter-group. To evaluate the effectiveness of each gait features category, Artificial Neural Network (ANN), Support Vector Machine (SVM) and Naive Bayes classifier (NBC) are chosen as classifier. Results obtained demonstrated that for ANN classifier, fusion of basic and kinematic gait features due to intra-group normalization attained performance with 100% of accuracy outperformed others. As for SVM with polynomial kernel function, the finest performance specifically 100% accuracy is attained based on basic gait features from intra-group normalization whilst NBC achieved the best accuracy of 93.75% due to fusion of kinetic and kinematic gait features with intra-group normalization. Overall, the results obtained proven the ability of the machine classifiers in classifying gait pattern of PD from normal gait pattern with basic spatiotemporal seems to be the most reliable feature for this purpose due to its superb performance that achieved during classification by the three classifiers.
Metadata
Item Type: | Thesis (Masters) |
---|---|
Creators: | Creators Email / ID Num. Manap, Hany Hazfiza 2010790279 |
Divisions: | Universiti Teknologi MARA, Shah Alam > Faculty of Electrical Engineering |
Programme: | Master of Science |
Keywords: | Parkinson disease; Machine learning approach; Parkinson gait |
Date: | December 2013 |
URI: | https://ir.uitm.edu.my/id/eprint/18311 |
Download
![[thumbnail of TM_HANY HAFIZA MANAP EE 13_5.pdf]](https://ir.uitm.edu.my/style/images/fileicons/text.png)
TM_HANY HAFIZA MANAP EE 13_5.pdf
Download (117kB)
Digital Copy
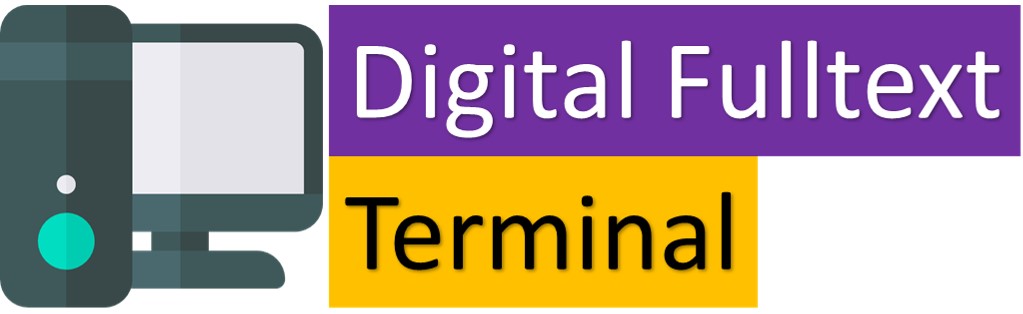

Physical Copy

ID Number
18311
Indexing
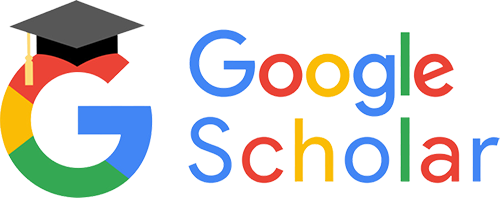
