Abstract
Due to the increasing population in our societies, the accurate identification of individuals has become crucial. As a result, the concept of access control has gained significance. Currently, the Automatic Fingerprint Identification System (AFIS) is the predominant method used for access control in restricted areas like immigration borders, labs, offices, and even smart devices. However, despite its widespread use, AFIS is highly vulnerable to presentation attacks involving the fabrication and presentation of fake fingerprints to AFIS. Efforts have been made to address this concern through hardware and software-based approaches. Hardware-based methods incorporate additional sensors to capture other live human traits during fingerprint authentication, such as pulse rate, blood flow, and odor. Unfortunately, attackers have found ways to create thin layered spoofs that can deceive these systems. As a result, software-based methods have emerged, which focus on learning inherent live fingerprint features to distinguish against spoofs.
Metadata
Item Type: | Thesis (PhD) |
---|---|
Creators: | Creators Email / ID Num. Ametefe, Divine Senanu UNSPECIFIED |
Contributors: | Contribution Name Email / ID Num. Thesis advisor Sarnin, Suzi Seroja (Ir. Ts. Dr.) UNSPECIFIED |
Divisions: | Universiti Teknologi MARA, Shah Alam > College of Engineering |
Programme: | Doctor of Philosophy (Electrical Engineering) |
Keywords: | Attack, fingerprint, detection |
Date: | 2023 |
URI: | https://ir.uitm.edu.my/id/eprint/89329 |
Download
![[thumbnail of 89329.pdf]](https://ir.uitm.edu.my/style/images/fileicons/text.png)
89329.pdf
Download (206kB)
Digital Copy
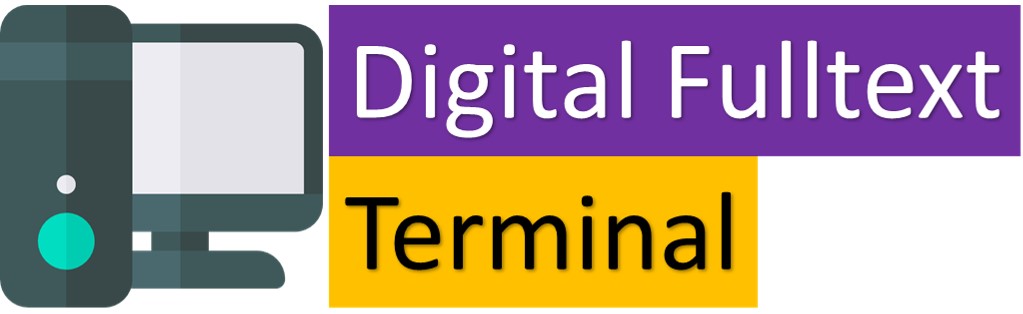
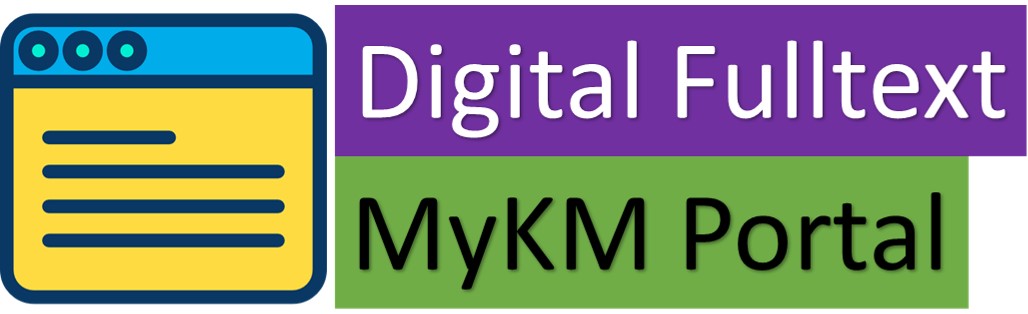
Physical Copy
ID Number
89329
Indexing
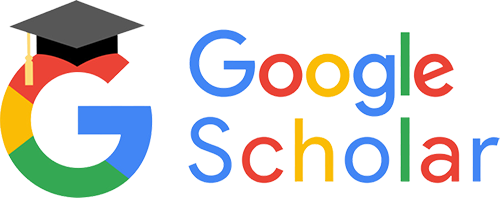
