Abstract
This project presents an artificial neural network ANN technique for predicting the output from a Grid-Connected Photovoltaic (GCPV) system. In this study, the ANN model utilizes solar irradiance (SI), ambient temperature (AT) and module temperature (MT) as it inputs while the output is the total AC power produced from the grid connected PV system. These data was collected from rooftop of Malaysian Green Technology Corporation (MGTC), Bandar Baru Bangi, Malaysia along January and October 2010. The main objective of this research is to predict AC kWh output from grid-connected photovoltaic system referring to its performance indicator. The indicators consist of root mean square error (RMSE) and coefficient of determination (R ), which is for checking the goodness of fit. The performance of ANN model was tested using different algorithm and activation function. The number of neuron has been varied from 1-20 while the momentum rate and the learning rate varies from 0.05 until 1. Levenberg-Marquardt shows the best fit training algorithm.
Metadata
Item Type: | Thesis (Degree) |
---|---|
Creators: | Creators Email / ID Num. Nor Adzman, Nurul Khairaini UNSPECIFIED |
Contributors: | Contribution Name Email / ID Num. Thesis advisor Sulaiman, Shahril Irwan UNSPECIFIED |
Divisions: | Universiti Teknologi MARA, Shah Alam > Faculty of Electrical Engineering |
Programme: | Bachelor of Electrical Engineering (Hons.) |
Keywords: | GCPV, artificial, neural |
Date: | 2013 |
URI: | https://ir.uitm.edu.my/id/eprint/84492 |
Download
![[thumbnail of 84492.pdf]](https://ir.uitm.edu.my/style/images/fileicons/text.png)
84492.pdf
Download (150kB)
Digital Copy
Physical Copy

ID Number
84492
Indexing
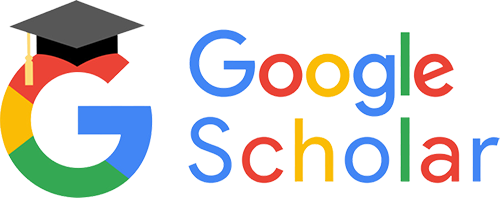
